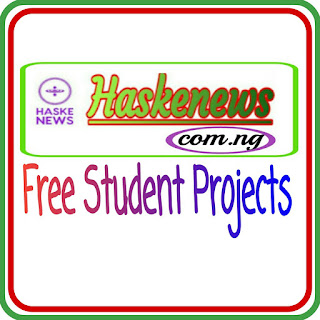
TIME SERIES ANALYSIS OF CRUDE OIL PRICE IN NIFERIA
Monday, July 20, 2020
Comment
CHAPTER ONE
1.0 Introduction
Crude Oil varies greatly in appearance depending on its composition. It is usually black or dark brown (although it may be yellowish, radish, or even greenish). In the reservoir it is usually found in association with natural gas, which being lighter forms a gas cap over the petroleum, and saline water which, being heavier than most forms of crude oil, generally sinks beneath it. Crude oil may also be found in semi-solid form mixed with sand and water, as in the Athabasca oil sands in Canada, where it is usually referred to as crude bitumen. (Oil Sands-Glossary, 2007).
Today, about 90 percent of vehicular fuel needs are met by oil. Petroleum also makes up 40 percent of total energy consumption in the United States, but is responsible for only 1 percent of electricity generation. Petroleum’s worth as a portable, dense energy source powering the vast majority of vehicles and as the base of many industrial chemicals makes it one of the world’s most important commodities. Viability of the oil commodity is controlled by several key parameters, number of vehicles in the world competing for fuel, quantity of oil exported to the world market (Export Land Model), Net energy Gain (economically useful energy provided minus energy consumed), political stability of oil exporting nations and ability to defend oil supply lines. (CBN, 2014).
The petroleum industry generally classifies crude oil by the geographic location it is produced in (e.g. West Texas Intermediate, Brent, or Oman), its API gravity (an oil industry measure of density), and its sulfur content. Crude oil may be considered light if it has low density or heavy if it has high density; and it may be referred to as sweet if it contains relatively little sulfur or sour if it contains substantial amounts of sulfur. The geographic location is important because it affects transportation costs to the refinery. Light crude oil is more desirable than heavy oil since it produces a higher yield of gasoline, while sweet oil commands a higher price than sour oil because it has fewer environmental problems and requires less refining to meet sulfur standards imposed on fuels in consuming countries. Each crude oil has unique molecular characteristics which are understood by the use of crude oil assay analysis in petroleum laboratories. Barrels from an area in which the crude oil’s molecular characteristics have been determined and the oil has been classified are used as pricing references throughout the world. Some of the common reference crudes are:
West Texas Intermediate (WTI), a very high-quality, sweet, light oil delivered at Cushing, Oklahoma for North American oil.
Brent Blend, comprising 15 oils from fields in the Brent and Ninian systems in the East Shetland Basin of the North Sea. The oil is landed at Sullom Voe terminal in Shetland. Oil production from Europe, African and Middle Eastern oil flowing west tends to be priced off this oil, which forms a benchmark.
Dubai – Oman, used as benchmark for Middle East sour crude oil flowing to the Asia – Pacific region.
Tapis (from Malaysia, used as a reference for light Far East oil).
Minas (from Indonesia, used as a reference for heavy Far East oil).
The OPEC Reference Basket, a weighted average of oil blends from various OPEC (The Organization of the Petroleum Exporting Countries) countries.
Midway Sunset Heavy, by which heavy oil in California is priced
Western Canadian selects the benchmark crude oil for emerging heavy, high TAN (acidic) crudes. (Natural Resources Canada (May 2011)).
1.1 Background of the Study
The crude oil industry in Nigeria is the largest industry and main generator of Gross Domestic Product (GDP) in the West African nation which is also the continent’s most populous. Oil is major source of energy in Nigeria and the world in general oil being the mainstay of the Nigerian economy plays a vital role in shaping the economic and political destiny of the country. Although Nigerians oil industry was founded at the beginning of the century, it was not until the end of the Nigeria civil war (1967-1970) that the oil industry began to play a prominent role in the economic life of the country (OPEC ASB, 2014).
Nigeria is a mono product economy because oil accounts for over 95 percent of its export earnings. Moreover, about 70 percent of government revenue is derived from oil and over 90 percent of new investments are associated with oil and allied products. As an oil-exporting third world nation, Nigerians economic development has witnessed trials and tribulations, as the Nations fortunes have risen and fallen in the strong seas of the international oil market. Nigeria’s vulnerability to oil price shocks stems from the nations over dependence on crude oil export. This is amply evident from the drastic decline in non-oil exports over the past three decades of petroleum production in Nigeria. (Huntington, 1994)
According to Oil and Gas Journal (OGJ), Nigeria had an estimated 36.2 billion barrels of proven oil reserves as of January 2009. Before the constriction of oil refinery at Port-Harcourt in 1964 oil produced was exported in crude form. Since the oil refinery in part-Harcourt could not meet the needs of both internal consumption and exports, oil refineries were constructed in Warri (1967) and Kaduna (1976) and with this export rose from 245,000 tons in 1958 to about 108 million tonnes in 1974.
A survey conducted in 1997 by environmental resources managers ltd, revealed that Nigeria has 159 total oil fields and 1481 wells in operation. The most productive region of the nation is the Coastal Niger Delta Basin in the Niger Delta or “South-South” region which encompasses 78 of the 159 oil fields most of Nigeria’s oil fields are small and scattered, and as of 1990, these small fields accounted for 62.1% of all Nigerian production. This contrasts with the sixteen largest fields which produced 37.9% of Nigeria’s petroleum at that time. As a result of the numerous small fields, an extensive and well-developed pipeline network has been engineered to transport the crude oil accounted for 7.1 percent of total exports in 1961, which was dominated at that time by cocoa, groundnut and rubber, in that order. In 1965 oil had climbed to 13.5 percent of the nation’s export earnings, and by 1970, it has become the leading source of foreign exchange accounting for 63.9 percent. The 1973 Arab oil embargo against the United States of America did not only earn Nigeria the windfall revenue of an oil boom. By 1979, petroleum sales had completely overshadowed non-oil exports, as it then contributed about 95 percent of the country’s export earnings. During the peak of the oil boom, Nigeria’s premium crude, the Bonny Light (37 API) fetched the commanding price of 40 dollars a barrel. In 1990, following the Gulf war and the United Nations trade embargo on Iraq and Kuwait, not only did the Organization of Petroleum Exporting Countries (OPEC) re-allocate the production shares of both nations to other producers such as Nigeria. There was also sharp momentary increase in crude oil prices. From the low spot price of 15.49 dollars in June 36.78 dollars a barrel in August 1990. In 1993, the spot price of the Bonny light, on the average, was about 18 U.S dollars per barrel. As of 2000 oil and gas exports accounted for more than 98% of export earnings and about 83% of federal government revenue, as well as generating more than 14% of its GDP. It also provided 95% of foreign exchange earnings and about 65% of government budgetary revenues.
1.2 Statement of the Problem
Constant fluctuations of oil price in Nigeria have been a matter of concern and it effect on macroeconomics variable which are determinant of economic growth. Crude oil has generated great wealth for Nigeria, but its effects on growth of the Nigeria economy as regards to returns and productivity is still questionable (Odularu, 2007). Recent hike in the price of crude oil products such as petrol, kerosene, diesel etc. has been linked to strong and increasing demand for crude oil particularly from emerging market economies. Hence, there is need to evaluate the relative impact of crude oil prices on the economic growth in Nigeria.
Above all, the research consequently intends to use the Box-Jenkins methodology to model and accommodate the dynamics of conditional heteroscedasticity in crude oil price data. Moreover by finding appropriate models to fit the data, the study also, intends to use them to predict future values based on the past observations.
1.3 Aim and Objectives of the Study
The aim of this research is to study the pattern and behavior of the quarterly prices of Nigerian crude oil using Box-Jenkins methodology to analyze crude oil price data. To achieve this, the following objectives are formulated:
To conduct a preliminary check on the data obtained to gain sight on the pattern of trend and seasonal components
To develop Autoregressive Integrated Moving Average (ARIMA) models for the crude oil prices.
To identify the model that best fit the data under study.
Use the model to forecast future crude oil prices.
To make recommendation based on our findings.
1.4 Significance of the Study
This research is significant to the extent to Nigerian society because the study is intended to inform the petroleum industry and the Nigerian leadership through developing models that can be used to forecast future crude oil prices, and thus, guide policy makers in formulating macroeconomic policies. Besides, the research would also add to volume of the existing literature and the future in the area.
1.5 Scope and Limitation
Box and Jenkins (methodology, 1976) is the basis of this study work; due to its power and flexibility in model fitting. The application in the study is limited to the quarterly prices of crude oil in Nigeria from 1986 to 2015.
1.6 Justification of the Study
The Nigerian economy is largely oil-dependent as it (oil) accounts for a significant proportion of the Gross Domestic Product. Also the structure of exports in Nigeria shows the acute dominance of this natural resource. This dominance is further revealed especially with regards to revenue generation by the government. Budgetary allocations are many a time made based on projections about the expected path of oil prices thus making the economy susceptible to volatility emanating from the international oil market. The sustained increases in oil price since the early twenty first century has provided Nigeria with huge financial resources which could be channeled in to productive use for boosting growth and development. This study therefore would contribute immensely to knowledge and total understanding of the economy in Nigeria, as it relates to the natural resource (crude oil). It will help the government and the general public to know the trend of oil prices. (Orubu, 2003) CHAPTER TWO
LITERATURE REVIEW
2.0 Introduction
Although the internet regarding the study of oil price forecasting and the impact of global economy is not new in the last decade the literature in the field has been booming based on researches and practitioners acting. Since crude oil is a commodity with huge strategic importance to all countries, there is a large consensus that higher oil price and volatilities impact global economic growth and financial markets (Hamilton, 2009). Moreover, the behavior of crude oil price has sparked interest of many practitioners and researchers among which we have drawn from their words. This chapter is therefore designed to review the theoretical issues behind economic time series and Crude oil prices.
2.1 Empirical Evidence
This study is focused on forecasting of crude oil price using a time series model. There are many studies that addressed the accuracy of crude oil price modeling and time series forecasting. Some of which are;
Yeldu and Muktar (2010) found that if the current trend continues, the production, export and domestic consumption of Nigeria’s state crude oil will be on the increase with 1006402, 838476 and 167926 thousand barrels expected by the year 2015 for production, export and domestic consumption respectively.
Coppola (2008) investigates the long- run relationship between repot and futures oil using weekly WTI spot and futures prices trend or the NYMEX from January, 1986 to September 2006. The author performs consecration tests and VECM to examine short and long-run relationships between spot and future prices. The in sample forecasting result indicate that futures price seem to explain sport price movements and the out of sample forecast results suggest that the VECM out performs thousand on walk model.
Fong and See (2002) apply a Markov regime switching (MES) model to explain the volatility of oil price. This model is based on the standard ARCH/GARCH approach, allowing jumps in the conditional variance between regimes. They use WTI crude oil daily prices for the nearest future contracts. Covering the period of January 2, 1992 to December 31, 1997. The results suggest that the regime switching model with the ARCH effects (RSARCH) outperforms the constant various and the standard GARCH. Model for the all three out of sample forecasts.
Wldon and Chamberlin (1933) in the process of determining oil price, OPEC studies the production cost of member’s state countries especially the marginal cost (MC) and marginal revenue (MR) curves. The summation of MR and MC curves of individual countries equals the cartel’ state MR and MC curves.
Oil price movement may also have an impact on stock prices. While there is a huge literature concerning the links between oil prices and economic activity, the literature relating to the relationship between oil prices and stock markets is rare. There is however a growing interest for this relation, mainly due to the increasing financial integration process. The impact of oil price movements on share prices has notable been investigated by Jones and Kaul (1996), Sandorsly (1999) or el- Sharif et al (2005). The seminal work by Jones and Kaul (1996) puts forward that oil prices impact the stock market, through their influence on expected dividends and cash-flows. Using a YAR framework, Sadorsky (199) also concludes to the existence of an of price influence on share prices.
Huntington (1994), structural forecasting models based on supply and demand were not successful in predicting oil prices in the 1990 due to two major errors. The first error was inaccurate forecasts of GDP, especially for developing countries and the second was an incorrect prediction of the increase in the supply of oil by non-OPEC countries.
Lanza et al., (2005) estimate the relationship between 10 heavy crude oil prices series and 14 petroleum product price series in Europe and United States. The study cover the period from 1994-2002, and the authors apply contegration and error correction (ECT) tests to determine the relationships among the variable and forecast crude oil prices. The empirical results provide evidence that product prices are related to heavy oil prices in the short term and long term. Furthermore, in the united State neither the error correction model (ECM) or the naive model dominates, whereas in Europe the ECM marginally outperforms the naïve model. Wang et al., (2005) apply ARIMA to model the linear component of monthly WTI crude oil data from January 1970 to December 2003. The out of sample forecasts indicate that the linear ARIMA model exhibits poor forecasting power when compared to the nonlinear integrated fuzzy expert system approaches.
Xie et al., (2006) forecast WTI crude oil prices by applying the ARIMA model to WTI spot prices from January 1970 to December 2003. They compare the result with those of support vector machine and artificial neural networks methods. Once again the out of sample forecasts indicate that the ARIMA model provides the poorest forecasting performance among the methods considered.
Fernandez (2010) performs an out of sample forecast for short and long term horizons employing daily natural gas and Dubai crude oil price from 1994- 2005 using an ARIMA model. The results indicate that for very short horizon forecasts, the ARIMA model outperforms the artificial neural networks and the support vector machine approaches, however, for long horizon forecasts, the ARIMA model underperforms the other approach.
Zeng and Swanson (1998) examine the forecasting ability of future prices on spot prices. For this purpose, several models are applied including random walk with drift, random walk without drift, VAR model and VECM models. They apply the models to daily future prices on four commodities including:
Crude oil traded on the NYMEX,
Gold traded on the new York commodity exchange,
Teasing bound traded on the Chicago board of trade, and
The s &p 500 index traded on the Chicago mercantile exchange for the period of April 1, 1990 to October 31, 1995. The results induced that the ECM model outperforms the other models.
Lalonde et al., (2003) investigate the effects of the non-oil variable on real WTI crude oil spot [prices. They use quarterly data from 1974-2001. In their model real WTI crude oil spot price if function of the world output gap and the real U.S dollar effective exchange rate gap. In addition, Their dummy variable are included in the model, including the 1979 Iranian revolution the Iran- Iraq war of 1980, the mid 1980s collapse of OPEC discipline, and the oil price collapse of 1986. They exclude the real U.S dollar effective exchange rate gap from the model since it is not found to be a significant variable, though they do include petroleum inventory levels. The out of sample forecasting results indicate that this model outperform the random walk model and the autoregressive model benchmarks. However, when inventory levels are excluded from the model, the forecasting ability is inferior to that of the two benchmarks.
Kulkarni and Haidar (2009) use a multilayer feed neural network (FNN) model to perform short term crude oil pre tendency forecasting and investigate the efficiency of future prices on spot price. They use daily WTI crude oil spot prices from 1996- 2007 and prices for 1-2-3amd 4 mouth WTI future contracts. They conclude that future prices provided new information about spot prices especially in the case of 1 and 2 out future cataracts.
Yu et al., (2005) propose a rough set refined year mining (RSTM) approach as a new knowledge based forecasting system for crude oil price tendency forecasting. This system is a combination of two modules; the first one applies the next mining technique to produce rough knowledge and the second one applies the rough set theory as knowledge refines for the rough knowledge. They use mouthy crude oil data from January 1970 to October 2004. The authors compare the out of sample forecasting ability of the RSTM approach to the random walk, the linear regression model the ARIMA model and the back propagation neural network (BPNN) model. He hit ratio of each model indicates that the performance of the new RSTM approach is better than the other models and the random walk model has the poorest performance.
2.2.0 Conceptual Framework
2.2.1 Crude Oil and the Nigerian Economic
Oil was discovered in 1956 at Oloibiri in the Niger delta after half a century of exploration, the discovery was made by shell BP, at the time the sole concessionaire, Nigeria joined the ranks of oil producers in 1953 when its first oil field came on stream producing 5,00 barrels per day after 1960, exploration right in onshore and offshore areas adjoining the Niger Delta were extended to other foreign companies. In 1965 the EA field was discovered by shell in shallow water southeast of Warri.
In 1970, the end of the Biafran war coincided with the world oil price, and Nigeria was able to reap instant riches from its oil production. Nigeria joined the organization of petroleum exporting countries (OPEC) in 1971 and established the Nigeria National Petroleum Company (NNPC) in 1977, a state owed and controlled company which is a major player in both the upstream and downstream sector (Blair, 1976).
While the discovery of oil in the eastern and mind western regions of the Niger delta pleased hopeful Nigerians, giving them an early indication soon after independent economic development was within reach at the sometime it signaled a danger of grave actually “burned” the country. This tension reached its peak with the civil war that lasted from 1967 to 1970. As the war commenced, the literature reflected the hostility, the impact and fate of the oil industry.
The U.S. remains the largest imports of Nigerians crude oil according for 40% of the country is total oil exports. Nigeria provides about 10% of overall U.S. oil imports and ranks as the fifth largest source for oil imports in the U.S.
There are several studies on the crude oil price that have taken the approach of Hamilton (1983) in investigating the effect of oil price shocks on levels of gross domestic product, noted that in spite of the considerable attentions of researchers on the presumed macroeconomic consequences of crude oil prices shock there is no consensus as to the transmission mechanism.
Ubogy (1984) sought to establish the impact of oil on the Nigeria economy. Examining the growth and developments of oil industry, government participation and the stages of the development of the industry, government revenue, foreign exchange earnings, employment generation and industry linkages effects, he noted that oil has been responsible for the radical increase in revenue and further buttressed the stronger dependence on oil revenue as envisaged in our development plans due to the unanticipated decline in oil earnings.
Obadan (1983), sought to evaluate the impact of the development in the oil sector on the Nigerian economy through, government finance, he found that effect of oil on government revenue is positive. That is, there is a positive relationship between oil price and government expenditure.
2.2.2 Crude Oil Price Economic Outputs
Although a number of empirical studies on the relationship between crude oil price and economic growth exist, most of such studies have largely focused developed economies. Very little imperial literature exists on net oil importing economies in sub-Sahara Africa. More so, very little exist on an emerging oil exporting countries in addition to these errors, Tang and Hammoudeh (2002) state that another source of error was the omission of market participant’s expectations of OPECs intervention.
Kaufman et al., (2004) investigate the impact of OPEC behavior on real oil prices. The author examine Ciranger causality between OPEC members cheating on quotas and the days of forward consumption of OECD crude oil stocks, calculated by dividing OECD crude oil stocks by OECD crude oil demand.
Change and Wong (2003) examined the effects of oil price fluctuations on the Singaporean economy and found an in significant negative relationship between oil price shocks and Singapor’s gross domestic output inflation and unemployment rate. On the contrary, the study by Farzanegan and Marwardt (2009) on the Ganian economy shows a strong positive relationship between oil price changes and industrial output growth. Also, studies by Wakeford (2006) and Bouzid (2012) have all found a negative relationship between crude oil price and economic growth.
In Nigeria, a few scholars have studied the impact of oil and stock prices on the Nigeria economy. For instance Ayadi (2000) examined he effect of crude oil production shocks on the net oil exporting country using standard VAR which includes crude oil production, output real exchange rate and inflation over 1975-1992 period, the impact responses shows that positive crude oil production shock was followed by rise in output, reduction in inflation and depreciation of domestic currency. In similar study, Olamide and Adejumo (2006) examined the effects of crude oil price shock on output, inflation, real exchange rate and money supply in Nigeria’s economy within the VAR framework they found no substantial role of crude oil price shocks in explaining movements in output and inflation, but on the long-run money supply and real exchange rate are significantly affected following a shock to crude oil prices.
Ahmad (2000), in his study found that there is unidirectional causal relationship between stock prices and investment spending in the case of India and Bangladesh. The major of researchers for developed countries agree upon the negative relationship between crude oil and economic growth. Empirical findings for developing countries vary more in directions of impacts. Different model specification, choice of variables and monetary policies can be the reasons of variations in results.
In light of the importance of crude oil to the world’s economy, it is not surprising that economists and statisticians have devoted great efforts towards developing methods to forecast price and volatility levels. While the most popular forecasting approaches are based on traditional econometrics, computational approaches such as artificial neural networks (ANN) and fuzzy experts system have gained popularity in financial markets because of their flexibility and accuracy. However there is still no general consensus on which methods are more reliable.
In this study, we make use of the quantitative method of QY price forecasting which utilizes numerical variables that impact oil prices, that can be further segmented into econometric model that are sorted into three classes of models. They are time series models financial models and structural model. But in the course of this work only the time series model is required for the forecasting of crude oil prices.
CHAPTER THREE
METHODOLOGY
3.0 Introduction
This chapter is designed to explain in detail the theoretical framework and research methodology employed in this study. Hence the various materials and methods applied for the purpose of achieving the objectives of the study are going to be explained thoroughly below. In particular, the basic procedures of the Box-Jenkins methodology and the Volatility clustering are explained in details.
3.1 Basic Definition and Concepts
Time series unlike the other statistical procedures such as the analysis of random sample of observations, is based on the assumption that successive values in data file represent consecutive measurements taken at equally spaced time intervals.
There are two main goals of time series analysis (1) identification of the nature of the phenomenon represented by the sequence of observations, and (2) forecasting (predicting future values of the time series variable). Both of these goals require that the pattern of observed time series data is identified and more or less formally described. Once the pattern is established, we can interpret and integrate it with other data (i.e., use it in our theory of the investigated phenomenon, e.g., seasonal commodity prices). Regardless of the depth of the understanding and the validity of our interpretation (theory) of the phenomenon, we can extrapolate the identified pattern to predict future events. (Yakubu, 2016).
Generally, time series is a sequence of data points, measured typically at successive points in time spaced at uniform time intervals. Examples of time series include the daily closing price of a company stock, monthly rainfall data, and yearly sales figures. Time series are very frequently plotted via line charts. Time series are used in statistics, signal processing, pattern recognition, econometrics, mathematical finance, weather forecasting, earthquake prediction, electroencephalography, control engineering, astronomy, and communications engineering. A time series, such as electric signals and voltage that can be recorded continuously in time is said to be continuous. On the other hand, a time series, such as interest rates, yield and volumes of sales that are taken only a specific time intervals, is said to be discrete. (Brockwell and Davis (2002)).
There are obviously, numerous reasons to record and to analyze the data of a time series. Among these are the wishes to gain a better understanding of the data generating mechanism, the prediction of future values or the optimal control of a system. The characteristic property of a time series is the fact that the data are not generated independent and identically, there dispersion varies in time, they are often governed by a trend and they have cyclic components.
In most other statistics analysis, it is assumed that in time series analysis, the data consists of a systematic pattern (usually a set of identifiable components) and random noise (error) which usually makes the pattern difficult to identify. Most time series analysis techniques involve some form of filtering out the noise in order to make the pattern more salient.
3.1.1 General Patterns of Time Series
Most Time Series patterns can be described in terms of two basic classes of components; trend and seasonality. The former represents a general systematic linear or (most often) nonlinear components that changes over time and does not repeat or at least does not repeat within the time range captured by our data. The later may have a formally similar nature. However, it repeats itself in systematic intervals over time. These two classes of time series components may coexist in real life data. Other patterns that are seen in time series analysis are cyclical and irregular. (Brockwell and Davis (2002)).
Trend Analysis
There are no proven “automatic” techniques to identify trend components in the time series data, however, as long as the trend is monotonous (consistently increasing or decreasing) that part of data analysis is typically not very difficult. If the time series data contain considerable error, then the first step in the process of trend identification is smoothing. A straight line or nonlinear curve can approximate trend of a time series, such as registration of numbers of vehicles. A linear trend equation is used to represent a time series data that can be increasing or decreasing by equal amount from one period to another.
Seasonality Analysis
Seasonal dependency (seasonality) is another general component of the time series pattern. It is formally defined as correlation dependency of order k between each ith element of the series and the (i-k)’th element and measured by autocorrelation (i.e., a correlation between the two terms); k is usually called the lag. If the measurement error is not too large, seasonality can be visually identified in the series as a pattern that repeats every k elements.
Seasonality component exhibits a short-term pattern that recurs seasonally. The analysis of a seasonal variation over period of time can also be useful in evaluating current figures. There are several ways of analysing a time series in order to isolate the seasonal variation. The most popular one is the method of moving average. The moving average method is used in measuring the seasonal fluctuation of a time series. It can also be used to smooth out fluctuations by moving the mean value through the data.
Cyclical Analysis
This type of component is very common with data on business and economic activities. It consists of a period of prosperity followed by periods of recession, depression and recovery in that order. An important example of cyclical variation is what is called business cycles. In business and economic activities, if variations recur after yearly intervals, then they are considered cyclical.
Irregular Analysis
Irregular component or variation in time series refers to the odd movements of a time series, which are due to chance. Events that may lead to such odd movements include industrial actions, earthquakes, floods, outbreak of epidemics and many more. Irregular variation is a combination of episodic and residual (chance) variations. Episodic variation, through unpredictable, can be identified. For example, the effect of an earthquake on a national economy could easily be brought to bear, but earthquake itself could not have been predicted. Residual variation, on the other hand, is unpredictable and cannot be identified.
3.1.2 Approaches to Economic Modeling and Forecasting
Broadly speaking, there are five approaches to economic modeling and forecasting based on time series data:
Exponential Smoothing Methods
Single-Equation Regression Models
Simultaneous – Equation Regression Models
Vector Autoregression (VAR) Models
ARIMA Models
The publication by Box and Jenkins of Time Series Analysis: Forecasting and control ushered in a new generation of forecasting tools. Popularly known as the Box – Jenkins Methodology, but technically known as ARIMA, the emphasis of these methods is not constructing single–equation or simultaneous–equation models, but on analyzing the probabilistic, or stochastic, properties of economic time series on their own under the philosophy ‘let the data speak for themselves’.(D.N. Gujarati 2010).
3.2.0 Time Series Models
3.2.1 White Noise Process
A time series { } is called a white noise process denoted by, if the following conditions are satisfied:
( i.e. Zero mean)
(i.e. constant variance)
Cov { , } = 0 if i.e. not serially correlated in this case we write
.
3.2.2 Autoregressive Process AR (p)
The auto regressive process uses weighted time lagged (previous) values to generate new current values for the time series. A time series { } is an AR (p) process if it has the following representation.
(3.1.1)
(3.1.2)
For where {} is a series of independent identically distributed (iid) random variables, and is some constant.
3.2.3 Moving Average Process MA (q)
The moving average technique is often used for linear fitting. A moving average process of order q denoted by (MA) q is a stationary time series process { } by box and Jenkins (1976), if it has representation of the form
(3.1.3)
(3.1.4)
3.2.4 Autoregressive Moving Average Process ARMA (p, q)
According to Newbold and Grangee (1974), an ARMA (p, q) process is defined from the combination of the order autoregressive and order moving average process. A time series { } is an ARMA (p, q) process if it has a representative form of
(3.1.5)
Where { }, is some constant, and the and are defined as for AR and MA models respectively and is a series of unknown random errors (white noise) which are assumed to follow the normal probability distribution. An ARMA process is stationary if the AR component of the series is stationary and invertible if the MA component is invertible.
3.2.5 Autoregressive Integrated Moving Average Process ARIMA (p, d, q)
This process was developed to help remove trends and uncover hidden patterns in non-stationary data because; ARMA process can only model stationary data. Although the theory behind ARIMA time series model was developed much earlier, the systematic procedure for applying the technique was documented in the landmark book by box and Jenkins (1970). Since the ARIMA forecasting and box and Jenkins forecasting usually refer to the same set of techniques.
Knowing that stationary time series is integrated of the order d and if by differencing the terms it becomes an ARIMA (p, d, q) process, then the difference process can have an ARIMA (p, d, q) representation. In this case the time series can be expressed as
(3.1.6)
Where
The important point to note is that to use the Box–Jenkins methodology, we must have stationary time series or a time series that is stationary after one or more differencing’s. The reason for assuming stationary is; the objective of = B-j (Box–Jenkins) is to identify and estimate a statistical model which can be interpreted as having generated the sample data. If this estimated model is then to be used for forecasting, we must assume that the features of this model are constant through time, and particularly over future time periods. Thus, the simple reason for requiring stationary data is that nay model which is inferred from these data can itself be interpreted as stationary or stable, therefore providing a valid basis for forecasting. (Michael, 1987).
3.2.6 Autoregressive Conditional Heteroscedasticity
The ARCH process introduced by Engle (1982) and Tim Bollerslev (1986) explicitly recognized the difference between the unconditional and the conditional variance allowing the latter to change over time as a function of past errors.
Let be a random variable drawn from the conditional density function Eagle (1982) proposed the following model:
, {} ~ iid N(0,1) (3.1.7)
Where is the positive function of {} defined by
(3.1.8)
With and
The simplest is the ARCH (1) process given by
(3.1.9)
is a sequence of identically and independently distributed random variables each with mean zero and unit variance and is independent of adding the assumption of normality, it can be more directly expressed in terms of the information set available at time t. Using conditional densities,
The innovation is presented to have unit variance so that the conditional variance of
(3.2.1)
Problems with the ARCH (q) Model
Non stationarity may be generated due to long lag in the conditional variance equation.
How does one decide on the value of q? For very many series, ARCH processes with fairly large orders are required to capture the dynamics in the conditional variances.
The non-negativity constrains may be violated resulting in forecasting of negative variances.
The GARCH model by Bollerslev (1866) can get round these problems by offering a more parsimonious model (it avoids over fitting).
3.2.7 The GARCH Model
If an autoregressive moving average model (ARMA model) is assumed for the error variance, the model is a generalized autoregressive conditional
heteroscedasticity (GARCH) model, Bollerslev (1986). In that case, the GARCH (p, q) model (where p is the order of the GARCH terms, is the order of the ARCH terms given by
(3.2.2)
Generally, when testing for heteroscedasticity in econometric models, the best test is the white noise test. However, when dealing with time series data, this means to test for ARCH errors (as described above) and GARCH errors.
3.2.8 Testing for Stationarity in a Time Series Data
A time series data is said to be stationary if its underlying generation process is based on a constant mean and constant variance with its Autocorrelation function (ACF) essentially constant through time. Thus, if different subsets of a realization are considered (time series ‘sample’) the different subsets will typically have means, variances and autocorrelations that do not differ significantly.
A statistical test for stationarity is the most widely used Dickey – Fuller test. To carry out the test, estimate by OLS the regression model:
(2.2.3)
Where is the differenced series . The number of term in the regression, p, is usually set to be about 3. Then, if is nearly zero the original series needs differencing and if < 0 then is already stationary. (Bhar and Sharma (2010)).
3.3 The Box–Jenkins (BJ) Methodology
Looking at a time series, such as the Oil Price series, how does someone know whether it follows a purely AR process (and if so, what is the value of p) or a purely MA process (and if so, what is the value of q) or an ARMA process (and if so, what is the value of p and q) or an ARIMA process in which case we must know the values of p, d, and q. Box-Jenkins methodology comes in handy in answering the preceding question. The method consists of four steps:
Step1: Model Identification
In model identification, Chatfield (1980) wrote that, the classes of time series models are selected that may be appropriate for a given observed series. After the plots and other necessary statistical computation are made from the data (crude oil price), and applying the knowledge on the topic of the research. We then, select a model that is appropriate for the data. However the three commonly used tools graphical methods in identification in time series are: - the time plot of the series, the time plot of autocorrelation at various lags (ACF) and the time plot of the partial autocorrelation function (PACF).
Graphical Analysis (Time plot of a series):- before one pursues formal test, it is always advisable to plot the time series under study. Such plots give initial clue about the likely nature of the time series.
Autocorrelation Function (ACF):- one simple test of stationarity is based on the so-called Autocorrelation Function.
The ACF at lag k, denoted by is defined as
Where covariance at lag k and variance of the process are defined as:
is the covariance (or auto covariance) at lag k, is the covariance between
.
Partial Autocorrelation Function (PACF):- the partial autocorrelation function (), measure correlation between (time series) observations that are k times period apart after controlling for correlations at intermediate lags (i.e. lags less than k). In other words, partial autocorrelation is the correlation between after removing the effect of the intermediate y’s.
Table 3.1: Theoretical Patterns of ACF and PACF
Model
ACF
PACF
White Noise
All zeros
All zeros
AR(p)
Exponentially Decay
p significant lags before dropping to zero
MA(q)
q significant lags before dropping to zero
Exponentially Decay
ARMA(p,q)
Decay after qth lag
Decay after pth lag
Source: L.M. Bhar and V.K. Sharma (2010).
Step2: Model Estimation
The unknown parameters of the preliminary ARIMA (p, d, q) model should be estimated, we consider criteria such as least square method and maximum likelihood in finding the best possible estimates of those unknown parameter within a given model
Step3: Diagnostic Checking
After estimation of the model, the Box-Jenkins model building strategy entails a diagnosis of the adequacy of the model more specifically, it is necessary to ascertain in what way the model is adequate and in what way it is inadequate.
A good way to check the model adequacy an overall Box-Jenkins model is to analyze the residual obtained from the model. In spite of this we can also check for model adequacy by examining the sample autocorrelation function of the residual (ACF) and the sample partial autocorrelation function of the residual (PACF). We can conclude that the model is adequate if there are no spikes in the ACF and PACF, we can also employ the Jarque–Bera test for non-normality of residual.
Fig 3.1: Box–Jenkins Methodology
Step4: Forecasting
The above four techniques model building processes typically repeated several times until a satisfactory model is finally selected for one to forecast. One of the reasons for the popularity of the ARIMA modeling is its success in forecasting. In many cases, the forecasts obtained by this method are more reliable than those obtained from the traditional econometric modeling, particularly for short – term forecasts (D. N. Gujarati, 2010). In time series, forecasting is a mathematical way of estimating future values using present and historical values of the series (Gujarati, 2010). Forecasting as described by Box-Jenkins (1976) provides basis for economic and business planning, inventory and production control and optimization of industrial processes. The efficiency validation of the considered models was evaluated by means of the following measures;
i) Root Mean Square Error (RMSE)
The RMSE is a measure of how well the model fits the data. It is defined as:
RMSE = (3.2.7)
Where the are the values of the predicted variable when all samples are include in the model formation, and is the number of observations. RMSE
ii) Mean Absolute Error (MAE)
The MAE is a quantity used to measure how close predictions are to the eventual outcomes.
(3.2.8)
It is an average of the absolute errors. i.e., where is the prediction and is the true value.
iii) Mean Absolute Percentage Error (MAPE)
The MAPE is a measure of prediction accuracy of a forecasting method in statistics. It usually expresses accuracy as a percentage, and is defined by the formula:
(3.2.9)
Where, is the actual value and is the forecast value.
The difference between and is divided by the Actual value again. The absolute value in this calculation is summed for every forecasted point in time and divided by the number of fitted points. Multiplying it by 100 makes it a percentage error
3.4 Information and Model Selection Criteria
In statistical modeling, one of the main challenges is to select a suitable model from a candidate family to characterize the underlying data. Model selection criteria provide useful tools in this regard, selection criteria assess whether a fitted model offers an optimal balance between goodness–of–fit and parsimony. Ideally, a criteria will identify candidate models that are either too simplistic to accommodate the data or unnecessarily complex. The most common model selection criteria are the AIC (Akaike (1974, 1976)), HQC (Hannan – Quinn (1979)), and SIC (Schwarz (1978)).
3.4.1 Akaike Information Criterion (AIC)
AIC is an important and leading statistics by which we can determine the order of an autoregressive model. Mr. Akaike developed this statistics according to his name this statistics is known as Akaike Information Criterion (AIC). The AIC takes into account both how well the model fits the observed series and the number of parameters to be used in the fit.
Let be the maximum likelihood of a model with k parameters based on a sample of size n. The information criteria for selecting the most parsimonious correct model are:
Where, the parameter bears the usual meaning. Akaike also mention that the minimum AIC criterion produced a selected model, which is hopefully closer to the best possible choice.
3.4.2 Hannan-Quinn Information Criterion (HQC)
In statistics, the Hannan-Quinn information criterion (HQC) is a criterion for model selection. It is an alternative to Akaike information criterion (AIC) and Bayesian information criterion (BIC) given as; Let be the maximum likelihood of a model with k parameters based on a sample of size n. The information criteria for selecting the most parsimonious correct model are:
Where k is the number of parameters, n is the number of observations.
3.4.3 Schwartz Information Criterion (SIC)
In 1978 Schwartz discussed a criterion denoted by SIC which help in deciding the order of auto regression. Initially he developed this criterion for taking decisions about the regress subset. Later Engel et al., in 1992 use this criterion as a tool for determining the order of auto regression and they defined this criterion as below:
Since the Schwarz information is derived using Bayesian arguments, this criterion is also known as the Bayesian Information Criterion (BIC). These criteria take the general form
Where in Akaike case, in Hannan – Quinn case in the Schwarz case. Using these criteria, a model is selected that corresponds to:
Where, the parameters bear the usual meaning. Schwartz also shows that this criterion is better than AIC. The model with minimum SIC assumes to describe the data series adequately. The minimum value of this criterion is desirable for the adequacy of a model.
3.5 Unit Root Test
Recently, testing for unit root has already became a standard procedure in time series studies and the application of unit root test such as Augmented Dickey-Fuller (ADF) and the Phillips-Perron (PP) are the most popular tests within it. Unit root test is importance because of the importance of stationary, the absence of unit root indicates that the series has some variances are not be determined by time and that effects shocks dissolve over time. Besides that, the existence of non-stationary variables will cause a spurious regression which has high R2 and t-statistic is significant but the results would not consist of any economic meaning. There are other tests for stationarity such as KPPS, Portmanteau test, LM test for autocorrelation and Jarque-bera test for normality.
3.5.1 Kwiatkowski-Phillips-Schmidt-Shin (KPSS) Test
Kwiatkowski-Phillips-Schmidt-Shin (1992) proposed a test of the null hypothesis that an observable series is trend stationary (stationary around a deterministic trend). The integration properties of a series may also be investigated by testing the null hypothesis that the series is stationary against a unit root. Assuming no linear trend term, the data generating process is given as:-
Where a random is walk, and is a stationary process. Kwiatkowski (1992) proposed the following test statistic KPSS Where With and an estimator of the long run variance of (3.3.6)
The null hypothesis of the test is against the alternative hypothesis . Reject the null hypothesis if the test statistic is greater than the asymptotic critical values.
3.5.2 Augmented Dickey Fuller (ADF) Test
The ADF regression equation due to Dickey and Fuller (1979) is given by: (3.3.7)
For
Where is the intercept, represents the trend in case it is present, is the coefficient of the lagged dependent variable. And p lags of with coefficients are added to account for series correlation in the residuals the null hypothesis is that the series has unit root while the alternative hypothesis is that the series is stationary. The ADF test statistics is given by:-
ADF Where is the standard error for , and denotes estimate. The null hypothesis of unit root is accepted if the test statistic is greater than the critical values.
3.6 Residual Analysis
In the test for residual there are various test including portmanteaus test, Lagrange multiplier, autocorrelation, partial autocorrelations, Jarque-Bera test, and Ljung –Box test, all test for the adequacy of the model.
3.6.1 Jarque-Bera Test
Jarque and Bera (1987) have proposed test for normality based on skewness and kurtosis of a distribution. The Jarque-Bera test is a two-sided goodness of fit test suitable when a fully-specified null distribution is unknown and its parameters must be estimated. The test statistic is given by
Where n is the sample size, s is the sample skewness, and k is the sample kurtosis. The test checks the pairs of hypothesis; and That is, the distribution is symmetry and hence normal. And for the alternative hypothesis it implies that, the distribution is asymmetry and hence non-normal.
The null hypothesis is accepted if the test statistic is less then critical values, and rejected if the test statistic is greater than the critical values.
3.6.2 Portmanteau Test
In statistics, portmanteau tests, test whether any group of autocorrelations of a time series are different from zero. That is, it is a test used for investigating the presence of autocorrelation in time series. Among portmanteau test are both the Ljung-Box test and the (now obsolete) Box-Pierce test. The portmanteau tests are used to test the stationary of time series. The Ljung-Box test statistic is given as:-
(3.3.9)
Where, T = number of observation, S = number of coefficients to test autocorrelation, = autocorrelation coefficient (for lag k) and Q=portmanteau test statistic. If the sample value of Q exceeds the critical value of a chi-square distribution with s degrees of freedom, then at least one value of r is statistically different from zero at the specified significance level. The null hypothesis is:-
All lags autocorrelation are zero
Against the alternative hypothesis which is:-
At least one lag contains non-zero correlations. The null hypothesis is accepted if the statistic is less than the critical values, and rejected if the test statistic is greater than the critical values.
3.6.3 Box-Ljung Test
The Box-Ljung test (1978) is a diagnostic tool used to test the lack of fit of a time series model. The test is applied to the residuals of a time series after fitting an ARIMA (p, d, q) models to the data, the test examines correlations of the residuals. If the autocorrelations are very small, we conclude that the model does not exhibit significant lack of fit.
Ho: the model does not exhibit lack of fit
Ha: the model exhibits lack of fit
3.6.4 Normal Probability Plot
Normal probability plot is a way of learning whether it is reasonable to assume that the error terms are normally distributed. Here the basic idea behind any normal probability plot is that; if the data follow a normal distribution with mean and variance, then a plot of the theoretical percentiles of the normal distribution versus the observed sample percentiles should be approximately linear.
CHAPTER FOUR
DATA ANALYSIS
4.0 Introduction
Having explored the general theory of time series models in the preceding chapter, this chapter has prepared to get the requisite analysis; fits the techniques of Autoregressive Integrated Moving Average (ARIMA) family of models, developed by Box and Jenkins (1976), to the Crude Oil Price data courtesy of the Central Bank of Nigeria, with a view to achieve the pre-stated aim and objectives of this research work. However, the data empolyed in this study comprise of 120 quarterly observations of the Crude Oil Price in Nigeria spanning from 1986 to 2015. As such, the results were discussed and interpreted properly in this very chapter as follows:
4.1 Data Presentation
Base on this research work, Average Quartly Nigerian Crude Oil Price from 1986 to 2015 (in US dollars per barrel) was collected and analyzed in differents criteria.
4.2 Explore the Time Series
To identify the model of any time series data, one most make a guess as to the data generation process. In doing this, one most begin by plotting the time plot, ACF and PACF of the series.
4.2.1 Graphical Analysis
4.2.1.1 Graphical Time Plot of the original series
Fig 4.1: Time plot of the original data
From figure 4.1, the oil price has showed a fluctuationnal pattern, suggesting that the mean of the crude oil price has been changing over time in Nigeria (not constant) and variance is also changing with time. This means we have two sources of unstationary: non-constant variance and non-constant mean. The former can be removed by taking the log of the data while the later can be removed by differencing.
4.2.1.2 Time Plot of the first Difference of the Data
Figure 4.2 shows the time plot of the first difference of the original series. A close examination of the plot shows that the mean is stabilized by the first differencin operation but as expected there is still variability in the variance. This suggests examining the time plot of the log of the original data.
Fig 4.2: Time plot of the first difference of the data
4.2.1.3 Time Plot of the log of series
Fig 4.3: Time plot of the log of the data
Fig. 4.3 signifies that, the log transformation stabilizes the variance compared to the plot of the original data. This lead us to concluded that the Box-Jenkins modeling procedure can be applied with the log of the data.
4.2.1.4 Time Plot of the first differnce of the log of series
Fig 4.4: Time plot of the first difference of the log of the data
Fig 4.4 shows that, the first difference of the log of the data has constant mean and variance. This confirms our earlier observation that the Box-Jenkins procedure should be applied to the log of the data rather than anything else.
4.2.1.5 The ACF and PACF of the Log of the Data
After the preliminary analysis it has seen that the data can be model using Box-Jenkins procedure. The idea of this section is to examine the correlation structure of the data using ACF and PACF shown below. Fig 4.5: The Correlogram (ACF and PACF) plot of the log of the data
Fig 4.5 shows that ACF is exponentially decaying and the PACF is significant at lag 25 before dropping to zero. So, we suspect AR component.
4.2.1.6 The ACF and PACF of the first Difference of Log of the Data
Figure 4.6 has shown below that, the estimated ACF and PACF signifies there is no much difference in the pattern. Thus, the ACFs at lag 2, 5 and 26 seem statistically different from zero (at the 95% confidence limit), but at all other lags are not statistically different from zero i.e. the ACF decayed after lag 26. Also the PACF is statistically different from zero only at lag 2, 4, 5 and 28; all other lags are not different from zero (at the 95% confidence limits) i.e. the PACF decayed after lag 26. We therefore, confidently concluded that the model for the data is mixed with both AR and MA component.
Fig 4.6: The ACF and PACF plot of the first difference of log of the data
Also, both ACF and PACF plot deduced that the series is non-seasonal, since the pattern of the spikes when counting four are not the same because its quarterly data. Hence the series is non-seasonal but integrated so; the best model that will fit the data is ARIMA model.
4.2.2 Unit Root Test
The idea of this section is to do formal test of the order of integration of the data, to confirm whether the order of integration is of order one as suggested by the comparison from the time plot of the log of the data and first difference of the log of the data.
4.2.2.1 Unit Root Tests of the Log of the Data
Table 4.1 ADF and KPSS tests of the log of the data
TEST
TEST STATISTIC
CRITICAL/P-VALUES
ADF without constant
1.44536
0.9636
ADF with constant
-0.420129
0.9035
ADF with constant and trend
-2.03106
0.5837
KPSS without trend
2.19850
10% 5% 1%
0.349 0.462 0.736
KPSS with trend
0.35892
10% 5% 1%
0.120 0.148 0.216
KPSS (Test of Stationary)
H0: LCOP is stationary
Ha: LCOP is non-stationary
From KPSS test, we observed that the data is not stationary before first difference because KPSS test statistic is greater than the critical value at 1%, 5% and 10% levels of significance, indicating that we rejected the null hypothesis (H0).
ADF (Unit Root Test)
H0: presence of unit root
Ha: No presence of unit root
Looking at our ADF p-values, we have strong evidence to fail to reject H0, which says there is presence of unit root in the data before first difference, i.e. we are accepting the null hypothesis since the p-values are greater than the value of alpha at 1%, 5% and 10% level of significance.
4.2.2.2 Unit Root Tests of the First Difference of Log of the Data
Table 4.2 ADF and KPSS tests of the first difference of log of the data
TEST
TEST STATISTIC
CRITICAL/P-VALUES
ADF without constant
-6.61961
1.544e-010
ADF with constant
-6.84123
8.815e-010
ADF with constant and trend
-6.82357
7.934e-009
KPSS without trend
0.0592112
1% 5% 10%
0.349 0.462 0.736
KPSS with trend
0.0465577
1% 5% 10%
0.120 0.148 0.216
KPSS (Test of Stationary)
H0: DLCOP is stationary
Ha: DLCOP is non-stationary
From KPSS test of stationary, we can concluded that the data is stationary at first difference because our KPSS test statistic is less than the critical value at 1%, 5% and 10% level of significance, indicating that we fail to reject H0, which claims stationary on the data at first difference.
ADF (Unit Root Test)
H0: presence of unit root
Ha: No presence of unit root
Looking at our ADF p-values, we have strong evidence to reject H0, which says there is no presence of unit root in the data at first difference, meaning we are rejecting the null hypothesis since the p-values are less than the value of alpha at 1%, 5% and 10% level of significance.
4.2.3 Further Examination of Periodicity
In order to look for periodic signals, there are several mathematical tools. A periodogram is one of them. A periodogram calculates the significance of different frequencies in time series data to identify any intrinsic periodic signals.
Fig 4.7: The plot shows the periodogram of the log of the data
The plot above shows the periodogram of the log of the data of quarterly crude oil price in Nigeria. It shows that there is one periodic signal or period in the data.
4.3 Model Identification
In the process of identify the model. The principle of parsimony is applied, as model used should be of the smallest number of parameters that will adequately representing the time series.
ARIMA (p, d, q), where p is the order of autoregressive process, d is the difference order of integration and q is the order of moving average process. By applying the principle of parsimony, and since after first difference, the data is said to be stable. Thus, d = 1, p,q = (0, 1, 2, 3, 4). 25 models were generated, out of which only 8 models met the requirement of being white-noise. As shown below;
4.3.1: Result of ARIMA model identification and selection for the Crude Oil Price series
Table 4.3: Result of ARIMA model identification and selection
MODEL
AIC
BIC
HQC
ARIMA(0,1,0)
-127.1139
-124.3348
-125.9854
ARIMA(0,1,1)
-132.1703
-123.8329
-128.7847
ARIMA(0,1,2)
-137.1327
-126.0162
-132.6186
ARIMA(0,1,3)
-140.3744
-126.4788
-134.7319
ARIMA(0,1,4)
-138.7283
-122.0536
-131.9572
ARIMA(1,1,0)
-126.8110
-118.4737
-123.4255
ARIMA(1,1,1)
-133.5014
-122.3849
-128.9874
ARIMA(1,1,2)
-140.0639
-126.1683
-134.4213
ARIMA(1,1,3)
-139.5828
-122.9080
-132.8117
ARIMA(1,1,4)
-138.2551
-118.8012
-130.3555
ARIMA(2,1,0)
-136.6304
-125.5139
-132.1164
ARIMA(2,1,1)
-136.7140
-122.8184
-131.0714
ARIMA(2,1,2)
-138.0643
-121.3896
-131.2833
ARIMA(2,1,3)
-139.3348
-119.8810
-131.4352
ARIMA(2,1,4)
-138.0561
-115.8231
-129.0280
ARIMA(3,1,0)
-135.8419
-121.9463
-130.1993
ARIMA(3,1,1)
-134.7794
-118.1046
-128.0083
ARIMA(3,1,2)
-136.5538
-117.0999
-128.6542
ARIMA(3,1,3)+
-140.1281
-127.8951
-135.1000
ARIMA(3,1,4)
-139.2167
-114.2046
-129.0601
ARIMA(4,1,0)
-138.8331
-122.1583
-132.0620
ARIMA(4,1,1)+
-141.5053
-121.0515
-132.6057
ARIMA(4,1,2)
-138.5057
-116.2743
-129.4792
ARIMA(4,1,3)
-139.7255
-114.7134
-129.5689
ARIMA(4,1,4)
-138.6054
-110.8142
-127.3202
Note:
+ = Best model
AIC = Alkaike information criteria;
BIC = Bayesian information criteria:
HQC = Hannan-Quinn information criteria.
Table 4.3 tested 25 models with low AIC, BIC and HQC which is common in ARIMA modeling and we have found the best models among them. ARIMA(3,1,3) and ARIMA(4,1,1) models are the best selected because they have minimum AIC, BIC and HQC. Therefore, ARIMA(3,1,3) in this case appeared to have minimum BIC as well as HQC while ARIMA(4,1,1) has minimum AIC.
4.4 Model Estimation
After the best models have been chosen, the parameters of the models are estimated. We considered Exact Maximum Likelihood as preference for the estimations. ARIMA (3,1,3 ) and ARIMA(4,1,1) are estimated by Gretl software using Kalman filter (exact ML). The dependent variable is the log of the data (l-COP) and the standard error based on Hessian. The results of these estimates are shown in the tables below:-
4.4.1: Result of ARIMA(3,1,3) model estimation of the Crude Oil Price data
Table 4.4: Result of ARIMA(3,1,3) model estimation for the Crude Oil Price
PARAMETER
COEFFICIENT
STD. ERROR
Z-STATISTICS
P-VALUES
Constant
0.0147738
0.00645397
2.289
0.0221 *
Phi_1
−0.0704481
0.206543
−0.3411
0.7330
Phi_2
0.0235844
0.156839
0.1504
0.8805
Phi_3
0.436059
0.173117
2.519
0.0118 *
Theta_1
0.305907
0.181121
1.689
0.0912 **
Theta_2
−0.362742
0.135621
−2.675
0.0075 ***
Theta_3
−0.616852
0.154596
−3.990
6.60e-05 ***
Mean dependent variable = 0.013672 S.D. dependent variable = 0.141251
Looking at our p-values, for the constant term phi_3 and theta_1 we are reject null hypothesis at 5% level of significance and conclude the parameters are statistically significant to the model, also phi_1, and phi_2 and are statistically insignificant there by accepting the null hypothesis, on the same way theta-2 and theta-3 are also statistically significant to the model there by rejecting the null hypothesis at 0.1% level of significance.
4.4.2: Result of ARIMA(4,1,1) model estimation of the Crude Oil Price data
Table 4.5: Result of ARIMA(4,1,1) model estimation for the Crude Oil Price
PARAMETER
COEFFICIENT
STD. ERROR
Z-STATISTICS
P-VALUES
Constant
0.0141468
0.00701551
2.017
0.0437 *
Phi_1
0.901963
0.235965
3.822
0.0001 ***
Phi_2
−0.561346
0.123988
−4.527
5.97e-06 ***
Phi_3
0.360934
0.131720
2.740
0.0061 **
Phi_4
−0.268370
0.0930777
−2.883
0.0039 **
Theta_1
−0.663952
0.243315
−2.729
0.0064 **
Mean dependent variable = 0.013672 S.D. dependent variable = 0.141251
From above table 4.5, the p-value for the constant term shows that we are rejecting the null hypothesis at 5% level of significance and concluded that it is statistically significant to the model, also phi-1 and phi-2 are all statistically significant to the model there by rejecting the null hypothesis at 0.1% level of significance, similarly phi-3, phi-4 and theta_1 are all statistically significant to the model there by rejecting the null hypothesis at 1% level of significance.
4.5 Model Checking
One of the assumptions for this is that, for a good model, the residuals must follow a white noise process, that is if the model fits the data well, the residuals are expected to be random; independent and identically distributed following the normal distribution (Bollerslev, 1986)
4.5.1 Residual Time Plots of the Data Using the Selected Models
Fig 4.7: Time plot of the residuals of ARIMA(3,1,3) model
Fig 4.7: Shows the time plot of residual of the data which can be obtained by subtracting the fitted l-cop from observed values. Moreover considering the plot, both mean and variance are stable, as the mean is towards zero and there is no much variation from the residuals, the variation is compacted.
Similarly, Fig 4.8: shows the time plot of residual of the data which can be obtained by subtracting the fitted l-cop from observed values. So, considering the plot, both mean and variance are stable, as the mean is towards zero and there is no much variation from the residuals, the variation is compacted.
Fig 4.8: Time plot of the residuals of ARIMA(4,1,1) model
4.5.2 Residual ACFs and PACFs of the Data Using the Selected Models
Fig 4.9: The residual ACF and PACF plot ARIMA (3,1,3) model
From Fig 4.9: we confirmed that there is no form of correlation amongst the residuals. Therefore, the model has passed the standard test criteria of being white noise, since the residuals are uncorrelated and stationary.
Fig 4.10: The residual ACF and PACF of ARIMA (4,1,1) model
Fig 4.10 confirmed that there is no form of correlation amongst the residuals. i.e. the model has passed the standard test criteria of being white noise, since the residuals are uncorrelated and stationary.
4.5.3 Unit Root Tests for the Residual of ARIMA (3,1,3) Model
Table 4.6 ADF and KPSS tests of the residual of ARIAM (3,1,3) model
TEST
TEST STATISTIC
CRITICAL/P-VALUES
ADF without constant
-10.6233
5.194e-065
ADF with constant
-10.5771
2.391e-015
ADF with constant and trend
-10.5441
3.347e-014
KPSS without trend
0.164577
1% 5% 10%
0.349 0.462 0.736
KPSS with trend
0.0912628
1% 5% 10%
0.120 0.148 0.216
KPSS (Test of Stationary)
H0: RESIDUAL1 is stationary
Ha: RESIDUAL1 is non-stationary
From the KPSS test we concluded that the residual of the data using ARIMA (3,1,3) model is stationary, because our KPSS test statistic is less than the critical value at 1%, 5% and 10% level of significance, indicating that we fail to reject H0, which claims stationary on the residual.
ADF (Unit Root Test)
H0: presence of unit root
Ha: No presence of unit root
Looking at our ADF P-values, we have strong evidence to reject the null hypothesis (H0), that is to say, there is no presence of unit root in the residual of the data using ARIMA (3,1,3) model, since the p-values are less than the value of alpha at 1%, 5% and 10% level of significance.
4.5.4 Unit Root Tests for the Residual of ARIMA (4,1,1) Model
Table 4.7 ADF and KPSS tests of the residual of ARIMA (4,1,1) model
TEST
TEST STATISTIC
CRITICAL/P-VALUES
ADF without constant
-11.0380
1.108e-078
ADF with constant
-10.9914
5.664e-016
ADF with constant and trend
-10.9779
8.197e-015
KPSS without trend
0.154695
1% 5% 10%
0.349 0.462 0.736
KPSS with trend
0.102205
1% 5% 10%
0.120 0.148 0.216
KPSS (Test of Stationary)
H0: RESIDUAL2 is stationary
Ha: RESIDUAL2 is non-stationary
From the KPSS test we concluded that the residual of the data using ARIMA (4,1,1) model is stationary, because our KPSS test statistic is less than the critical value at 1%, 5% and 10% level of significance, indicating that we fail to reject H0, which claims stationary on the residual.
ADF (Unit Root Test)
H0: presence of unit root
Ha: No presence of unit root
Looking at our ADF P-values, we have strong evidence to reject the null hypothesis (H0), that is to say, there is no presence of unit root in the residual of the data using ARIMA (4,1,1) model, since the p-values are less than the value of alpha at 1%, 5% and 10% level of significance.
4.5.5 Normality Test of the Residuals
In time series and econometric modeling, we often encounter the normality test as part of the residuals diagnosis to validate models assumptions. Normality tells us whether standardized residuals approximately follow a Gaussian distribution; this is normally determined by so many statistics and plots as shown below;
Fig 4.11 Normal probability plot of the residual of ARIMA (3,1,3) model
From the two figures above and below respectively, we observed that, the relationship between the theoretical percentiles and the sample percentiles is approximately linear. Therefore, the Normal Probability Plot of the residuals of the data using ARIMA (3,1,3) and ARIMA (4,1,1) models suggests that the error terms are indeed normally distributed.
Fig 4.12 Normal probability plot of the residual of ARIMA (4,1,1) model
4.5.5.2 The Jarque-Bera Test
The idea of this is to do formal test of the normality to confirm whether the error terms are indeed normally distributed.
Table 4.8: Result of Jarque-Bera test of the residuals
Jarque-Bera test
TEST STATISTIC
P-VALUE
ARIMA(3,1,3) model
3.456787
0.134008
ARIMA(4,1,1) model
3.113455
0.110233
From the Jarque-Bera test, we therefore accept the null hypothesis and concluded that, the error terms are purely random. Hence, there may not be any need to look for any other ARIMA model.
4.5.5.2 The Ljung-Box Test
By examine the correlations of the residuals of the ARIMA (3,1,3) and ARIMA(4,1,1) we concluded that the model does not exhibit significant lack of fit, since the autocorrelations are very small. It also justified from the p-values, which are all greater than the value of alpha at 1%, 5% and 10% level of significance, there by accepting null hypothesis.
4.5.5.3 ARCH Test for ARIMA (3,1,3) Model
Table 4.9: Test for ARCH of order 4 of ARIMA (3,1,3) model
Coefficient
std. error
t-ratio
p-value
alpha(0)
0.0134657
0.00445296
3.024
0.0031 ***
alpha(1)
−0.00581686
0.0953515
−0.06100
0.9515
alpha(2)
0.183443
0.0951106
1.929
0.0563 *
alpha(3)
−0.0547920
0.0952270
−0.5754
0.5662
alpha(4)
0.0156927
0.0949086
0.1653
0.8690
Test statistic: LM = 4.44169
with p-value = P(Chi-square(4) > 4.44169) = 0.349518
Since the p-value is greater than value of alpha, we therefore accepted the null hypothesis and concluded that no ARCH effect in the residual of the data using ARIMA(3,1,3) model.
4.5.5.4 ARCH Test for ARIMA (3,1,3) Model
Table 4.10: Test for ARCH of order 4 of ARIMA (4,1,1) model
Coefficient
std. error
t-ratio
p-value
alpha(0)
0.0150816
0.00446787
3.376
0.0010 ***
alpha(1)
−0.00917135
0.0951979
−0.09634
0.9234
alpha(2)
0.197012
0.0948385
2.077
0.0401 **
alpha(3)
−0.0757484
0.0949562
−0.7977
0.4268
alpha(4)
−0.0596805
0.0947792
−0.6297
0.5302
Test statistic: LM = 5.13491
with p-value = P(Chi-square(4) > 5.13491) = 0.273733
Null hypothesis: no ARCH effect is present
Since the p-value is greater than value of alpha, we therefore accept null hypothesis and conclude that no ARCH effect in the residual of the data using ARIMA(4,1,1) model.
4.6 Forecasting
Recall that the data ranges from 1986 to 2016 which we want to forecast crude oil price in Nigeria. To measure the forecasting ability, we have to estimate within sample forecasts. The purpose of forecasting within the sample is to test for the predictability power of the models selected, i.e. ARIMA (3,1,3) and ARIMA (4,1,1) as shown below;
4.6.1: Result of ARIMA (3,1,3) Forecasted Values for the Crude Oil Price Series (for 95% confidence intervals, z(0.025) = 1.96)
Table 4.11: Result of ARIMA (3,1,3) Forecasted Values for the Crude Oil Price (for 95% confidence intervals, z(0.025) = 1.96)
Observations
Prediction
std. error
95% interval
2016:1
4.535700
0.125166
4.290379 - 4.781022
2016:2
4.549167
0.198946
4.159240 - 4.939094
2016:3
4.560426
0.227385
4.114760 - 5.006092
2016:4
4.596311
0.245035
4.116052 - 5.076570
2017:1
4.608945
0.266363
4.086882 - 5.131007
2017:2
4.622834
0.279191
4.075631 - 5.170038
2017:3
4.646826
0.289676
4.079072 - 5.214580
2017:4
4.659996
0.301309
4.069441 - 5.250551
Table 4.12: Result Conversion of ARIMA (3,1,3) Forecasted Values for the Crude Oil Price Series (for 95% confidence intervals, z(0.025) = 1.96)
Observations
Prediction
std. error
95% interval
2016:1
93.29
1.133337
72.99 – 119.23
2016:2
94.55
1.220116
64.02 – 139.64
2016:3
95.62
1.255313
61.24 – 149.32
2016:4
99.12
1.277666
61.32 – 160.22
2017:1
100.39
1.305209
59.55 – 169.19
2017:2
101.78
1.322059
58.89 – 175.92
2017:3
104.25
1.335995
59.09 – 183.93
2017:4
105.64
1.351627
58.52 – 190.67
Fig 4.13: Time plot of actual and forecasted values of crude oil price data series of ARIMA (3,1,3) model
4.6.2: Result of ARIMA (4,1,1) Forecasted Values for the Crude Oil Price series (for 95% confidence intervals, z(0.025) = 1.96)
Table 4.13: Result of ARIMA (4,1,1) Forecasted values for the Crude Oil Price (for 95% confidence intervals, z(0.025) = 1.96)
Observations
Prediction
std. error
95% interval
2016:1
4.539672
0.126099
4.292523 - 4.786821
2016:2
4.577892
0.200678
4.184569 - 4.971214
2016:3
4.580832
0.230011
4.130019 - 5.031645
2016:4
4.584347
0.251465
4.091486 - 5.077208
2017:1
4.589790
0.268270
4.063991 - 5.115589
2017:2
4.591549
0.276825
4.048982 - 5.134116
2017:3
4.598579
0.282842
4.044220 - 5.152938
2017:4
4.612972
0.289737
4.045097 - 5.180846
Table 4.14: Result Conversion of ARIMA (4,1,1) Forecasted values for the Crude Oil Price Series (for 95% confidence intervals, z(0.025) = 1.96)
Observations
Prediction
std. error
95% interval
2016:1
93.66
1.134394
73.15 – 119.29
2016:2
97.31
1.222230
65.67 – 144.20
2016:3
97.60
1.258613
62.18 – 153.18
2016:4
97.93
1.285908
59.83 – 160.32
2017:1
98.47
1.307700
58.21 – 166.60
2017:2
98.65
1.318936
57.34 – 169.71
2017:3
99.34
1.326896
57.08 – 172.94
2017:4
100.78
1.336076
57.12 – 177.83
Fig 4.14: Time plot of actual and forecasted values of crude oil price data series of ARIMA (4,1,1) model
Fig 4.13 and 4.14 displayed the original and the forecasted values of the Crude Oil Price series in Nigeria produced by the ARIMA (3,1,3) and ARIMA(4,1,1) models, that is, from first Quarter, 2015 to fourth Quarter 2016. The figures also displayed how the forecasted values behave, which signifies that the models fitted the data well.
4.6.3 Model Validation Based on Forecasting Power
To measure the forecasting ability, we have to estimate within sample forecasts. The purpose of forecasting within the sample is to test for the predictability power of the models selected. If the magnitude of the difference between the forecasted and actual values is small, then the models have good forecasting power. A further relevant statistic is Theil’s U (Theil’s, 1966), define as the positive square root of U. the more accurate the forecasts, the lower the value of Theil’s U.
Table 4.15: Forecast Evaluation Statistics
ARIMA (2,1,4)
ARIMA (4,1,1)
Mean Error
-0.00077993
-0.00037549
Mean Squared Error
0.015781
0.016003
Root Mean Squared Error
0.12562
0.1265
Mean Absolute Error
0.093161
0.094115
Mean Percentage Error
-0.16067
-0.14207
Mean Absolute Percentage Error
2.7135
2.7372
Theil's U
0.88797
0.89751
In this case, both the two selected models have shown good result as evident from the above tables (with minimum MSE). One can observe that the forecasted series of ARIMA (3,1,3) model are closer to the accuracy series. Therefore, it can be concluded that the prediction power of ARIMA (3,1,3) model is better and suitable for quarterly periods forecasting, as such they best fit the data (Ngailo E. (2011)). That is to say the model that best fit the data is ARIMA (3,1,3) since it has the lowest forecast errors.
4.6.6 Discussion of Findings
The data set contains 120 observations from 1986 to 2015. Quarterly frequency is chosen for most explanatory factors. After which the data was subjected to various tests to choose the best model and forecast of the future oil prices was made and we concluded that prediction from our forecast shows a drastic increase in the crude oil price when it is compared to the previous records.
We assume that there exist some fundamental factors that contributed to the hike or fall of crude oil prices quarterly. In general crude oil fluctuation can be caused by supply and demand imbalance arising from a specific way, these markets functions as well as a number of peculiar events like changes in political regimes, economic crisis, trading tactics and so on.
CHAPTER FIVE
SUMMARY, CONCLUSION AND RECOMMENDATION
5.0 Introduction
This chapter summarizes the major findings of the study, present the conclusions and make recommendations based on the findings.
5.1 Summary
The Box-Jenkins four-step iterative methodology comprising of model identification, model fitting, model diagnostic and forecasting is applied to the Nigerian Crude Oil Price quarterly data between the periods 1986 to 2015. For the purpose of findings, a time series model that is optimal but for descriptions and prediction. The ARIMA (3,1,3) model turns out to be the best.
5.2 Conclusion
The study has presented us with an opportunity to have an extensive understanding of the theory of time series and its application to real life situation. The stages in the model building has been explored and utilized in the study. Based on the three information criteria AIC, BIC and HQC, the optimal time series models are ARIMA (3,1,3) and ARIMA (4,1,1). So far, the diagnostic criteria are concerned, but for the purpose of predictions, the best model is ARIMA (3,1,3). Hence, we concluded that prediction from our forecast shows a drastic increase in the crude oil price when it is compared to the previous records.
5.3 Recommendation
The findings are relevant for policy makers and industry analysts. It will be of great important if the Nigerian Petroleum Industry is extensively sensitized to eliminate whatever non-natural cause of the increase in the crude oil prices. Decision-making bodies in this industry however need to be systematic in finding a better market for the Nigerian crude oil by considering factors that may influence hike such as the coming back of the Islamic Republic of Iran on the list of petroleum exporters. Our findings could be helpful for monitoring oil markets and developing policies for stabilizing oil. With the knowledge of other factors, the forecast result can be used to achieve viable and workable framework on future price pattern.
References
Ahmad, G. (2000). On the Relation Between the Expected Value and the Volatility of the Nominal Excess Return on Stocks. The Journal of Finance. 48(5): 1779-1801.
Akaike, H. (1974). A New Look at the Statistical Model Identification. I.E.E.E. Transaction on Automatic Control, AC. 19: 716-723.
Ayadi, N.D. (2000). Effect of Oil Price on Net Oil Exporting in the United States. Energy. 21(1): 29-38.
Bhar, L.M. and Sharma, V.K. (2010). Time Series Analysis. New Delhi, India. Statistics Research Institute.
Blair, S.G. (2007). OPEC on the Approach of Globalization: An Event Study of Global Oil Prices. Global Economy Journal. Berkeley Electronic Press. 7(1): 234-267.
Bollerslev, T. (1986). Generalized autoregressive conditional heteroskedasticity, Journal of Econometrics. 31: 307-327.
Box, G. E. P., & Jenkins, G. M. (1976). Time Series Analysis: Forecasting and Control. San Franciso: Holden- Day.
Brockwell, P.J. and Davis, R.A. (2002). An Introduction to Time Series and Forecasting, (2nd Edition). Springer, New York: Springer University Press.
Central Bank of Nigeria (CBN), Annual Reports and Statement of Accounts – Various Years. (9.9 Central Bank of Nigeria, CBN (2014)): “The Structure of the Nigerian Economy and Implications for Development”, Realm Communication Ltd, Lagos.
Chatfield, C. (2003). The Analysis of Time Series: An Introduction Chapman & Hall/CRC.
Coppola, A. (2008). Forecasting Oil Price Movements: Exploiting the Information in the Futures Market. Journal of Futures Market. 28: 34–56.
Denis Kwiatkowski, P.C.B. Phillips, P. Schmidt, and Yongoheol, S. (1992). Testing the Null Hypothesis of Stationarity Against the Alternative of a Unit Root. Journal of Econometric. 54: 159-178.
Dickey, D.A.and Fuller, W.A. (1979). Distributions of the Estimators for Autoregressive Time Series with a Unit Root. Journal of the American Statistical Association. 74: 427-431.
Farnandez, C. (2010). A sample Approach to Short-term and Long-term Oil Price Forecasting. Energy Economics. 23: 325-338.
Farzanegan, L and Marwardt, R. (2009). On the Relation Between the Oil Price and Economic Growth. The Journal of Finance. 48(5): 1679-1791.
Fong, W.M. and K.H. See. (2002). A Markov switching model of the conditional volatility of crude oil prices. Energy Economics,Vol. 24: 71–95.
Gujarati, D.N. (2010). Economic Modeling and Forecasting Based on Time Series. Energy Economics. 23: 511-532.
Hamilton, J.D. (1983). Oil and Macro Economy Since World War II. The Journal of Political Economy. 91: 228-248.
Hamilton, J.D. (2009). Oil Prices and Volatilities Impact on Global Economic Growth. Energy Economics. 29: 387-409.
Hannan, E.J. (1980). The Estimation of the Order of an ARMA Process. Annals of Statistics. 8: 1071-1081.
Hannan, E.J., and B.G. Quinn, (1979). The Determination of the Order of an Autoregression. Journal of the Royal Statistical Society, B. 41: 190-195.
Huntington, H.G. (1994). Oil Price Forecasting in the 1980s: What Went Wrong?. The Energy Journal. 15(2): 1-22.
Jarque, C.M. and Bera, A.K. (1980). A Tests for Normality of Observations and Regression Residuals. International Statistical Review. 55(2): 163-172.
Jones, B. and Kaul, P. (1996). The impact of energy derivatives of the crude oil Market. Energy Economics Journal. 21: 135-167.
Kaufmann, R.K., Dees, S., Karadeloglou, P. and Sanchez, M. (2004). Does OPEC Matter? An Econometric Analysis of Oil Prices. The Energy Journal. 25(4): 67-90.
Kulkarni, P. and Haidar, N. S. (2007). Modeling Oil Price Volatility. Energy Policy. 35: 6459- 6563.
Lalonde, R., Z. Zhu, and F. Demers,. (2003). “Forecasting and Analyzing World Commodity Prices.” Renew of Financial Economics. 12: 2003–2024.
Lanza, A., M. Manera, and M. Giovannini. (2005). Modeling and Forecasting Cointegrated Relationships Among Heavy Oil and Product Prices. Energy Journal. 27: 831-848.
Michael, P. (1987). An Introduction to Economics. New York, Basil Blackwell P.343.
Newbold and Grangee. (1974). Time series Analysis and Forecasting: The Box- Jenkins Approach. Butterworth, London; Boston.
Obadan, E. (1983). Development in the Oil Sector on the Nigerian Economy. The Energy Economic Journal. 22: 105-127.
Odularu, K. (2007). Oil Shocks and the Macro Economy Role of Price Variability. The Energy Journal. 16: 39-56.
Oil Sands-Glossary. (2007). Mines and Minerals Act. Government of Alberta. Archieved from the Original on November 1, 2007. Retrieved October 2, 2008.
Olamide, B. and Adejumo, M. (2006). The Asymmetry of the Impact of Oil Price Shocks on Economic Activities: An Application of the Multivariate Threshold; Model Energy Economics. 27: 455-476.
Orubu, C.O. (2003). The Development and Contribution of the Petroleum Industry to the Nigerian Economy: Structure, Growth and Development. Mindex Publishers, Beninn-city.
Sadorsky, P. (1999) Oil Price Shocks and Stock Market Activity. Energy Economics Journal. 21(1): 29-38.
Ubogy, E. (1984). Impact of Oil on the Nigerian Economy. The Energy Economic Journal. 29: 405-427.
Wang, S.Y., L. Yu, and K.K. Lai. (2005). “A Novel Hybrid AI System Framework for Crude Oil Price Forecasting.” Lecture Notes in Computer Science. 3327: 233–242.
Wldon, S.S. and Chamberlin, H.C. (1993). Oil Prices and Real Exchange Rates. Energy Economics. 29: 390-404.
Xie, W., L. Yu, S. Xu, and S. Wang. (2006). “A new method for Crude Oil Price Forecasting Based on Support Vector Machines.” International Conference on Computational Science (Part IV), pp. 444–451.
Yakubu, M. (2016) Time Series Lecture Note (Unpublished). Department of Mathematics, Usman Danfodiyo University Sokoto, Nigeria.
Yeldu, E. and Muktar, A. (2010). Productivity, Export and Domestic Consumption of Crude Oil State in Nigeria. Energy Economics. 23: 511-532.
Yu, L., S.Y. Wang, and K.K. Lai. (2005). A Rough-set-refined Text Mining Approach for Crude Oil Market Tendency Forecasting. International Journal of Knowledge and Systems Sciences. 2: 33–46.
Zeng, T. and N.R. Swanson. (1998). Predective Evaluation of Econometric Forecasting Models in Commodity Future Markets. Studies in Nonlinear Dynamics and Econometrics. 2: 159–177.
0 Response to "TIME SERIES ANALYSIS OF CRUDE OIL PRICE IN NIFERIA"
Post a Comment