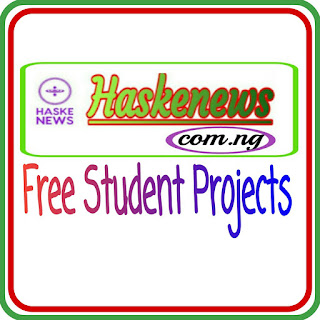
modification of ratio type estimator of the finite population mean under stratified random sampling
Monday, July 20, 2020
Comment
Background of the study
You May Also Like
- Understanding the application of multiple linear regression model in health care research pdf
- DEFAULT PREDICTORS IN AGRICULTURAL CREDIT SCORING: EVIDENCE FROM BANK OF AGRICULTURE DATA SOKOTO
- ANALYSIS OF SSCE EXAMINATION RESULT USING CANONICAL CORRELATION AND PRINCIPAL COMPONENT ANALYSES
- VOLATILITY MODELING OF DAILY EXCHANGE RATE BETWEEN UNITED STATES DOLLAR AND NIGERIA NAIRA
Auxiliary variables are commonly used in survey sampling to improve the precision of estimates. Whenever there is auxiliary information available, the researchers want to utilize it in the method of estimation to obtain the most efficient estimator. In some cases, in addition to mean of auxiliary, various parameters related to auxiliary variable, such as standard deviation, coefficient of variation, skewness, kurtosis, etc. may also be known (Koyncu and Kadilar (2009). In recent years, a number of research papers on ratio type and regression type estimators have appeared, based on different types of transformation. Some of the contributions in this area are due to Sisodiya and Dwivedi (1981), Upadhyaya and Singh (1999), Singh and Tailor (2003), Kadilar and Cingi (2003, 2004, 2006), Singh et.al.(2004), Khoshnevisan et.al. (2007), Singh et.al. (2007) and Singh et.al. (2008). In this article, we study some of these transformations and propose an improved estimator.
The problems of estimation of population parameters and search of the efficient estimators in present day have brought about rapid development in the theory and practice of sample surveys. The enumeration of population by sampling method proposed by Laplace in the later part of the eighteenth century came into wide spread use by Kiaer (1895) and Bowley (1906,1913) of England who were the leading users and advocates of sampling for obtaining social and economic data.
The purpose of a sample surveys is to collect information on a fraction of total population based on a random sample. The population here means collection of all the sampling units in a given region at a particular point of time or a particular period. For example, if the medical facilities in a hospital are to be surveyed through the patient registered in the hospital during the time period of survey will be the population. Similarly, if the production of wheat in a district is to be studied, then all the fields cultivating wheat in that district will constitute the population. The population may also refer to human population of the country. The information we want to get may be the total number of units, mean of various characteristics per units or the proportion of units which have certain characteristics.
In the theory of survey sampling, the auxiliary information is frequently used to increase the precision of estimators. In many such approaches and practices, the classical ratio, product and regression type estimators are widely used for estimating the unknown parameters, provided there exists a sufficient correlation between the study variable and the auxiliary variable. Auxiliary variable have been used by various authors in various estimation situation. Cochran (1940) introduced the use of an auxiliary variable at the estimation stage and proposed the ratio estimator for the population mean.It is well known fact that the ratio estimator ensures better efficiency than the sample mean estimator if the study variable and auxiliary variable have strong positive correlation. For situation when they are negatively correlated, the product estimator is also more efficient than the sample mean estimator. The product estimator was introduced by Murthy (1964). The ratio estimator provides better estimate of population parameter when the line of regression of variable of interest y on auxiliary variable x is linear and passes through origin, but in practical situation it is real so. Sometimes the line of regression of variable of interest y on auxiliary variable x is linear but may not pass through origin, in such situation, the difference and the regression estimator was introduced. It is well known that the regression estimators is more efficient than the sample mean estimator for
1.2 Aim and Objectives of the study
The aim of the present dissertation is to propose a modified ratio type estimator of the finite population mean under stratified random sampling to achieve the following objectives:
to derive the bias and the mean square error of the proposed estimator
to obtain the minimum mean square error of the proposed estimator
to make theoretical comparison with the existing estimators
to make numerical comparison with existing estimators using real life situation data.
1.3 Outline of the Study
Proposed outlines of the present dissertation work that will be discussed in various chapters are given below:
Chapter I: provides an introduction to the classical or traditional estimators, and uses of auxiliary information.
Chapter II: focuses on the review of some existing estimators in literature and some modified estimator such as modified ratio, regression and exponential estimator by several scholars.
Chapter III: devoted to the proposal of modified ratio estimator under stratified random sampling with mathematical derivation of expression for the bias and mean square error up to the first order of approximation. The optimum value of the parameters involved and minimum mean square error are to be derived.
Chapter IV: focuses on the theoretical and numerical comparison of the exponential estimator with some existing estimators available in literature.
Chapter V: Involves the discussions and conclusions.
2.1 Classical Estimators.
Let U = {U1,. . .UN} be a finite population of size N and the units are partitioned into K distinct strata with hth stratum containing Nh units (h = 1, . . . , K) such that
. Let a sample of size nh units (h = 1, . . . , k) be drawn from this population using a simple random sample preferably without replacement (SRSWOR). such that . Let (yhi, xhi) be the observed values of (y, x) on the ith unit of the hth stratum (i = 1, 2, . . . , Nh). Moreover, the population means of the variables y and x in the hth stratum are;
,, and the corresponding sample means of the variables y and x in the hth stratum are; ,.
The sample means of the variables y and x, in stratified random sampling, and is given by;
Where denotes the stratum weight. Also, are the unbiased estimators of the population means respectively.
We assume that the population mean and the population variance of an auxiliary variable are known. Let be the correlation coefficient between the study variable and the auxiliary variable. Also, assume and are the coefficient of variation between the study variable Y and an auxiliary variable X, and is the coefficient of variation between Y and X.
It is also well known that Sample mean estimator, it is unbiased and is defined as ;
and its Variance is given by;
Cochran (1940) advocated the use of additional information at estimation stage and discussed ratio type estimator. In ratio method of estimation, information on auxiliary variable is available which is linearly related to the variable under study and is utilized to estimate the population mean. When the correlation coefficient ρ between the study variable y and the auxiliary variable x is positive and high, it is advised to use the ratio method of estimation to estimate the population parameters of the study variate. The ratio estimator is more efficient than the sample mean under SRSWOR if
When ρ, the correlation coefficient between the two variables is nearly equal to the ratio of their coefficient of variations, then the ratio estimator will be as efficient as the regression estimator. The ratio estimator for estimating the population mean , is defined as;
Assuming the population mean is known.
The Bias and MSE of is given as;
Murthy (1964) presented product type estimator to estimate population mean while the study variable and auxiliary variable are negatively correlated. When the correlation coefficient ρ, between the study variable y and the auxiliary variable x is negative and high, then a complementary method to the ratio method of estimation usually known as product method of estimation is quite useful in estimating the population parameters of the study variable. This shows that if auxiliary information is such that; The product estimator is analogous to the ratio estimator and its theory and treatment are similar. Like ratio estimator, the product estimator is also biased. The product estimator for estimating the population mean , is defined as;
,
Assuming the population mean to be known.
The Bias and MSE of is given as;
Later on, another conventional estimator came into existence known as difference estimator which is similar to regression estimator. The difference estimator is an unbiased estimator for the population mean. When ρ, the correlation coefficient between the two variables is nearly equal to the ratio of their standard deviations, the difference estimator with k=1, will be as efficient as the regression estimator. The difference estimator for the population mean is defined as;
where k is a known constant.
The MSE of is given thus;
If the study variate y is approximately a constant multiple of an auxiliary variate x, it is more precise to estimate the population mean or population total by fitting a linear regression. Such an estimator is called a regression estimator. The regression estimator for the population mean is defined by;
and its MSE is given as;
2.2 The separate classical Estimators
It is well known that the variance of the stratified sample mean under SRSWOR is given by;
The separate ratio estimator for the population mean is defined as;
and its MSE is given as;
;
Where,
The separate product estimator for the population mean is defined as;
and its MSE is given as;
Also, the separate regression estimator for the population mean is defined as;
Here, denotes the sample regression coefficient of y on x in the ith stratum, where;
and its MSE is given as;
2.3 Some Existing modified Ratio Type Estimators in Stratified Random Sampling
Bahl and tuteja(1991) estimator in stratified sampling
The bias and MSE of t, to a first degree of approximation are given by
Sisodia and Dwivedi (1981) estimator is given by
The bias and MSE of are respectively given by
Singh and Kakran (1993) estimator is given by;
The bias and MSE of are respectively given by
Upadhyaya and Singh (1999) estimators is given by;
The bias and MSE of and are respectively given by
G.N. singh estimator (2001) using values of and and proposed the following two estimators
The bias and MSE of and , to a first degree of approximation are given by
And
Singh et.al.(2008) proposed the family of estimator given by,
The bias and MSE of , to a first degree of approximation are given by
References
CochranW.G. (1940).Theestimationoftheyieldsofthecerealexperimentsbysampling for the ratio of grain to total produce. Journal of Agricultural Science, Vol 59, 1225-1226
Murthy, M.N. (1964).Product method of estimation. The Indian Journal of Statistic A, Vol. 26,69-74
C. Kadilar and H. Cingi. (2003). Ratio estimators in stratified random sampling, Biometrica Journal. 45, pp. 218–225.
C. Kadilar and H. Cingi. (2005). A new ratio estimator in stratified random sampling, Comm. Statist. Theory Methods 34, pp. 597–602.
H. P. Singh and G. K. Vishwakarma. (2006). An efficient variant of the product and ratio estimators in stratified random sampling, Statistics in Transition 7, pp. 1311–1325.
Rao, T.J. (1991). On certain methods of improving ratio and regression estimator. Communication in statistics - Theory and Methods, Vol 20(10),3325-3340
Singh, R., Chauhan, P. And Sawan, N. (2008). On linear combination of ratio-product type exponential estimator for estimating finite population mean. Statistic in transition, Vol 9 (1), 105-115.
E.I. Enang, J.O. Uket, and J.E. Emmanuel (2017). A Modified Class of Exponential-Type Estimator of Population Mean in Simple Random Sampling. International Journal of Advanced Statistics and Probability, Vol. 5, No. 2, 70-76
G. O. Kadilar (2016). A New Exponential Type Estimator for the Population Mean in Simple Random Sampling. Journal of Modern Applied Statistical Method, Vol. 15, No. 2, 207-214.
Gupta S., and Shabbir J., (2012). Estimation of the mean of a sensitive variable in the presence of auxiliary information. Communications in Statistics-Theory and Methods, Vol. 41 (13),2394-2404
R. Tailor, B. Sharma, and J. M. Kim. (2011). A generalized ratio-cum-product estimator of finite population mean in stratified random sampling, Commun. Korean Statist. Soc. 18, pp. 111–118
Clement, E. P. & Enang, E. I. (2015). Calibration approach alternative ratio estimator for population mean in stratified sampling. International Journal of Statistics and Economics, 16(1), 83-93.
0 Response to "modification of ratio type estimator of the finite population mean under stratified random sampling"
Post a Comment