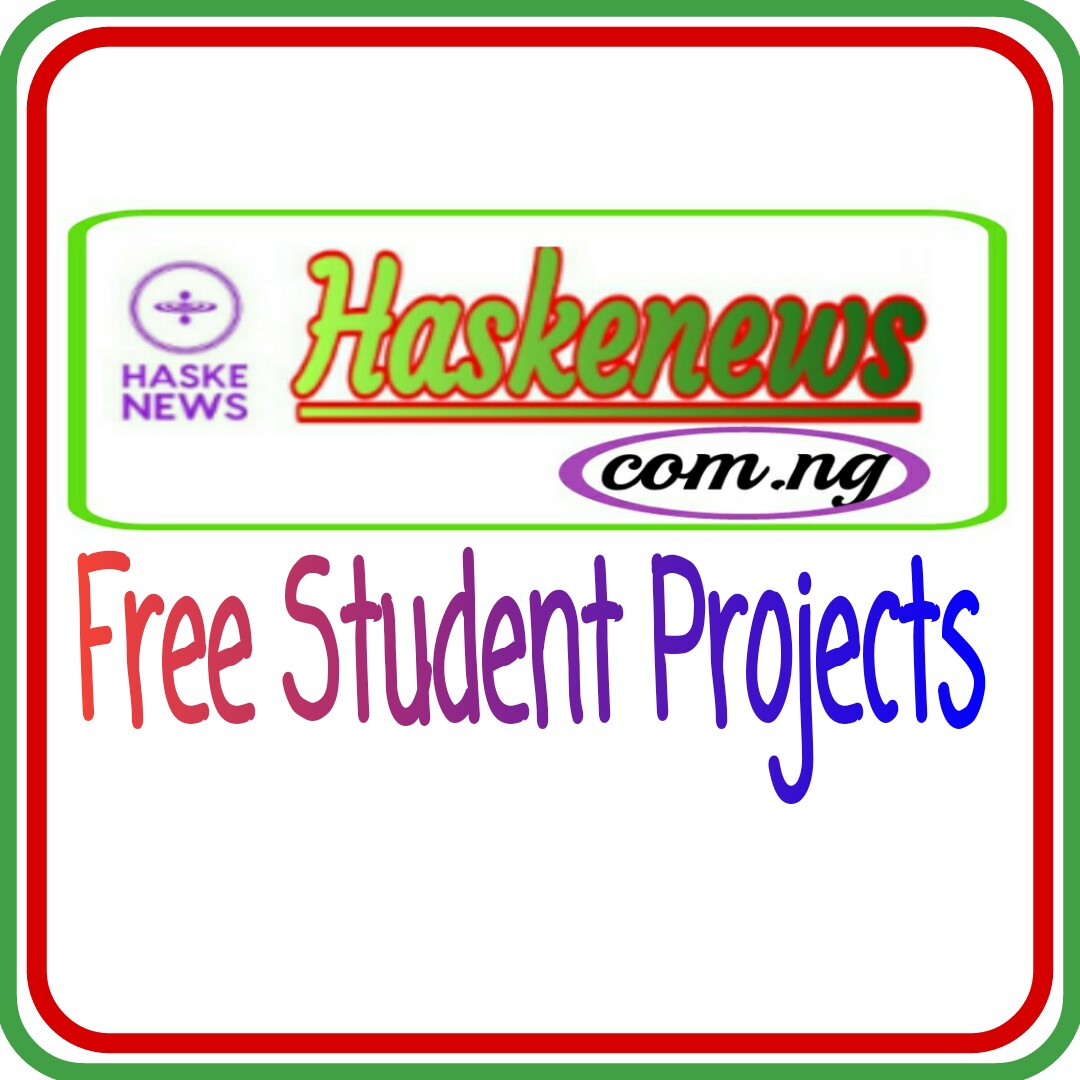
A MULTIVARIATE ANALYSIS OF VARIANCE (MANOVA) ON THE RESPONSE OF CROP PERFORMANCE TO FERTILIZER TYPES, PLOTS AND SOIL TYPES (A CASE STUDY OF NAJANU MEMORIAL FARMS KAURA NAMODA ZAMFARA STATE)
Friday, July 17, 2020
Comment
A MULTIVARIATE ANALYSIS OF VARIANCE (MANOVA) ON THE RESPONSE OF CROP PERFORMANCE TO FERTILIZER TYPES, PLOTS AND SOIL TYPES
(A CASE STUDY OF NAJANU MEMORIAL FARMS KAURA NAMODA ZAMFARA STATE)
BY
USMAN ABDULMALIK
ADM NO: 1310212018
A PROJECT RESESARCH SUBMITTED TO THE DEPARTMENT OF MATHEMATICS, STATISTICS UNIT IN PARTIAL FULFILMENT OF THE REQUIREMENT FOR THE AWARD OF BACHELOR OF SCIENCE (B.Sc) DEGREE IN STATISTICS KEBBI STATE UNIVERSITY OF SCIENCE AND TECHNOLOGY, ALEIRO.
OCTOBER, 2017
CERTIFICATION
This is to certify that this project work was carried out by USMAN ABDULMALIK with Admission number 1310212018 in the department of mathematics, statistics unit is fully adequate in scope and qualify for the award of Bachelor of Science (B.Sc) degree in statistics Kebbi state university of science and technology, Aliero. (KSUSTA).
Dr. Onwuka Gerald Ike Date
Project Supervisor
Dr. R. V. K. Singh Date
Head of Department
Prof R. A. Apinyomi Date
External Examiner
DEDICATION
This research project is dedicated first to the “Almighty Allah” for his enabling strength he bestowed on me in completing this work.
Secondly to my late brother Jamaluddeen Usman.
ACKNOWLEDGEMENT
First of all, I want to thank Allah for his mercy on me and the strength of reaching this stage of my study. And also my sincere gratitude goes to my supervisor, Dr Gerald .I Onwuka for his supervision, assistance and piece of advice throughout the compilation of this work. I pray Allah to recompense him abundantly.
I would like to extend my gratitude profoundly to all my lectures for the knowledge impacted on me that made this enviable feat, among which are Dr. Gerald .I Onwuka, Dr. R. V. K Singh, Dr. Babayemi A. W., Dr. Nweze, Mrs. T. O. James and all the lectures of the department I pray to Allah (S.W.T) to reward them all immensely.
My deepest love and gratitude goes to my father, ALH. Usman B. Umar kwasara for his encouragement, prayers and financial support through the course of this feat. I pray to Allah (S.W.T) to bless him with much more fruitful years in life and then crown him with Aljannat Firdausi in hereafter (Ameen). It is in this view that I most extend my profound and diligent gratitude to my mother HAJ. Khadija usman kwasara I pray to Allah (S.W.T) reward her immensely in the hereafter and may He Allah increase her in good deed and fruitful years (Ameen). And I equally appreciate the wonderful contribution of my beloved step mother HAJ. Hauwa`u Usman kwasara for her support and advice I pray may Allah (S.W.T) to always replenish them purse externally and make her also an inmate of Aljannat in hereafter (Amen). These dissertation would be incomplete if I failed to recognized my beloved brothers and sisters for your love and care may Allah continue to guide you until you reach beyond where I stand today (Ameen). And also my sincere gratitude to my friends and colleagues, among which are Emmanuel sule, Bashar Abubakar yabo, Abdulrahman Ibrahim (PROFSTA). Abubakar Abdullahi (GWANKS), Aisha Abdulmalik who guide me with good advice and helps in so many things during this wonderful program. And my deepest gratitude to whom their names are so many to be mentioned thank you all and love you all.
TABLE OF CONTENT
Title page……… ……. ……. …….. ……. ……….. ……… ……. …… ……. ……….. ………i
Certification……… ………. ……… ……….. ……. …… …… ….. ……… ……. ……….…...ii
Dedication………. ……… ……. …….. ….. ……… ……….. ……… …… ….. ……………...iii
Acknowledgement………… …………. ……….. …….. ………. ………. …….. ……………..iv
Table of content……… ………… …………… ……….. ………….. ……….. ………………..v
List of table of content……… ……… …….. ……… ……….. ……….. ………… …………...vi
List of table of content……….. ……….. ……….. ……….. …………. …………. …………..vii
List of table ……… ………. ………. ……….. ………. ……….. …………………………….viii
Abstract …… ……… …….. ……… …… …….. …… ……. …… …… ….. …. ….. ……….. ix
Chapter one
Introduction……….. ………. ………. ……….. ……….. ………. ……… …………..1-4
1.1 Statement of the problem. ………. …….. ………… ………. ……….. ………. …….4-5
1.2 Aim and objectives of the study…. ………. …….. ………… ………. ……….. ………5
1.3 Significance of the study…. ………. …….. ………… ………. ……….. ………. ……..5
1.4 Scope and limitation of the study…. ………. …….. ………… ………. ……….. ……..5
1.5 Definition of some basic terms …. …………… ………. ………. …….. …….. ……...5-6
Chapter two
2.0 Literature review …….. ……… …….. ………… ……… ……….. …..... ……………..7
2.1 Introduction… ……..…….. ……… …….. ………… ……… ……….. ……...... ………7
2.2 Review of some related literature ………………. …….. ……… …….. ………… …17-13
Chapter three
3.0 Methodology…….. ……… …….. ………… ……… …………………… ……………14
3.1 Introduction…….. ……… …….. ….......... …………………………………………….14
3.2 Research Design……… …….. ………… ……… ……….. ……............... ……………14
3.3 Data Description …….. ……… …….. ………… ……… ……….. ………… .......14
3.4 Multivariate Variables Specification…….. ……… …….. ………… ……… ……14-16
3.5 Statistical Methodology…….. ……… …….. ………… ……… ……….. ……… ...16-18
3.6 Hypothesis Testing In MANOVA…….. ……… …….. ………… ……… ………..18-19
3.6.1 Pillai’s Trace…….. ……… …….. ………… ……… ……….. ……. .............. ……….19
3.6.2 Hotelling- Lawley’s Trace…….. ……… …….. ………… ……… ……….. ……........19
Wilk’s Lamda (… ……..…….. ……… …….. ………… ……… ……….. ……20-21
3.6.4 Roy’s Largest Root… … ……… ………. …….……………………………………….21
Statistical package to be used for the study….. ……… ……… ……… ………………22
3.8 Data description…….. ………. ………. ……… ……… …….. ……… ……. …… ….23
3.9 Data analysis…….. ………. ……… ……… ……… ……… …….. …….. …….. ...23-30
Chapter four
4.0 Summary conclusion and recommendation. …….. ……… …….. ……………………..31
4.1 Summary……………………….. ………………… ……………………………………31
4.2 conclusions…….. ……… …….. ………… ……… ……….. …….................................31
4.3 Recommendation. …….. ……… …….. ………… ……… ……….. ……................31-32
4.4 Reference…….. ……… …….. ………… ……… ……….. ……..............................33-39
List of table
Table 3.9.1: Bartlett Test of Homogeneity of Variance…….. ……. ……… ……… …………..23
Table 3.9.2: MANOVA for plots……… ……… ………. ………. ………. ……… …….. 23-24
Table 3.9.3: MANOVA for soil types….. ……… …….. ……… ……… …….. ……. ……24-25
Table 3.9.4: MANOVA for fertilizer types……. ………… ………… ………….. ………...25-26
Table 3.9.5: MANOVA for interaction between plots and soil types…………… …………….26
Table 3.9.6: MANOVA for interaction between plots and fertilizer types…………………..26-27
Table 3.9.7: MANOVA for interaction between soil types and fertilizer types….. ………...27-28
Table 3.9.8: MANOVA for interaction between plots, soil types, and fertilizer types………28-29
Table 3.9.9: General ANOVA for crop performance versus plots, soil, and fertilizer………29-30
ABSTRACT
This study carried out to determine the response of fertilizer types, plots and soil types on crop performance by using multivariate analysis of variance (MANOVA). The data regarding the field courtesy of Najanu memorial farms was collected and analyze based on multivariate statistics such as wilk`s lambda. Pillai`s trace. Hotelling lawley`s and Roy`s criteria Hence the result confirmed that fertilizer types and any interaction with fertilizer types has significant effect on the crop performance, there by rejecting the null hypothesis at specified level of significance , whereas the remaining factors and the interaction has no significance effect on the crop performance. Thus, this signifies that fertilizer types have strong impact on the performance of crops. Therefore, future researchers should carry out a similar research work with more than three interaction effects to compare the results and also find out among the fertilizer types specifically which one has more effect.
CHAPTER ONE
INTRODUCTION
This research work is based on the multivariate analysis of variance (MANOVA) which is an extension of univariate analysis of variance (ANOVA). In ANOVA, differences among various group means on a single response variable are studied. In MANOVA, the number of response variables is increased to two or more. The hypothesis concerns a comparison of vectors of group means. Using univariate analysis, multiple ANOVA’s were conducted independently. The research works topic of study is response of crop performance to fertilizer types, plots and soil type which can be suitable to conduct this research using MANOVA since there are dependent and independent variables. When only two groups are being compared, the results are identical to Hotelling’s T2 procedure. The multivariate extension of F-test is not completely direct. Instead, several test statistics are available, such as Wilks’ Lambda and Lawley’s. Everitt et al.,(1991)
Draft animals are often in poor condition at the end of dry season, and many extension programmers have recommended that farmers grow and conserve fodder for their draft animals. On-station trials have demonstrated the potential for growing forage legumes and pasture grasses, but, to date, the production of single-purpose fodder crops at farm-level in West Africa has been minimal. In southern Mali, small quantities of forage cowpeas have been grown, because this was made a condition of credit allocation (Sangare, et al., 1988). Even in this case there has been a tendency to make this a dual-purpose crop, with a small harvest for human consumption (Starkey, 1988d). Crops cultivation is the most important section of agriculture, and the other section is husbandry or animal rearing. Agriculture in Nigeria has greatly improved in the past years because of the advent of technology and other necessary infrastructure.
Initially, most Nigeria farmers merely engage two types of farming, first one is subsistence agriculture that is to provide food for their family usage in the very little scale, and the second one is the commercial agriculture that is when the farmer has a very large scale farming made available in the market. Growth in agricultural output has no doubt been on the rise as farmer are stepping away from subsistence agriculture and embracing modern civilization-investing in large scale farming and ultimately increasing agricultural products. Soil fertility replenishment should be considered as an investment in the natural resource capital (Sanchez et al., (1997). reversing soil fertility depletion is one of the requirements for increasing per capital agricultural production in Africa (Sanchez and Leakey, 1997). Options aimed at improving soil fertility should rely on soil nutrient – supplying capacity, available soil amendments, and judicious use of mineral fertilizers to achieve balance nutrient management systems. Such an approach is usually referred to as integrated soil fertility management (ISFM) and should be embedded in a framework that includes aspects such as: weather, the presence of 7 weeds, pests and diseases, crop management, and socio-economic aspects such as input and output prices and labor availability(Bontkes and Wopereis, 2003 a).
The Nigeria soil and climatic condition is very suitable for the production of wide verities of crops, there are over a hundred different food crops produced by farmers in Nigeria on yearly basis which includes rice, yam, maize, okra, millet, sorghum, beans, potatoes, cassava, carrot, onion garbage, cocoa, cocoa yam, pears, onion, vegetables and very many others. Therefore, whatever type of cultivation a farmer wants to conduct, the knowledge of fertilizer and the type of soil is the most important in determining the farmer outputs. Soil and fertilizer type are very significant factor in crop production. According to food and agriculture organization of the united nation Nigeria is the world number 1 producer of cassava because of the topography of the soil structure and texture; cassava farming has taken the center stage in Nigeria and contributes over 45 percent of Nigeria agricultural Gross Domestic production (GDP). Agriculture in Nigeria contributes merely 24 percent of the Nigeria total GDP, trailing behind petroleum which is the major Nigeria domestic product. Although Nigeria depends heavily on the oil industry for its budgetary revenue it is believed that if the agriculture sector is properly managed and enhanced, it would greatly boost the country’s gross domestic product and even replace oil on the list, considering the vast area of land that is unused in Nigeria.
In many African countries including Nigeria, the main use of fertilizer is on maize, sorghum/millet and rice (Camara et al., 2006) with cowpea receiving little attention from farmers in terms of fertilizer application. As farmers lack adequate nutrient resources to fertilize all crops, they prefer to apply fertilizers to cereals and rarely target fertilizers directly to grain legumes which are mostly grown on residual fertility (Zingora et al., 2008). It is generally believed by most cowpea growers that the production of legumes do not require inorganic fertilizer application (Kan’’ankuk’’a, 1999). This is due to excessive vegetation at expense of grain production of this crop under fertilized fields.
In 1990, it was speculated that about 82 million hectares out of Nigeria’s total land area of 91 million hectares were farmed. Much of this land was formed under the bush fallow system, a process whereby land is left idle for a period of time to allow natural generation of soil fertility and replacement of soil nutrient. It is believed that the agricultural sector is one of Nigeria’s potential sources of revenue that is yet underdeveloped and unexplored.
Although mineral fertilizers can improve crop nutrition, they are sparingly used by farmers in Nigeria, as in many regions in sub-saharan Africa, partly due to the prohibitive cost as a result of removal of government subsidies (Gerner et al., 1995). Sanginga et al., (2009) stated that fertilizer consumption pattern within nations in Africa are often sketchy and inconsistent. Most smallholder farmers in Africa use fertilizers, but they are seldom able to apply them at the recommended rates and at the appropriate time because of high cost, lack of credit, delivery delays, and low variable returns (Heisey et al., 1996). Such constraints are largely due to the lack of enabling policy environment in rural areas caused by poor road and market infrastructure typical in most African countries. The price of fertilizers in rural areas of Africa is usually at least twice the international price (Bumb et al, 1996). African countries subsidized fertilizers; however, the removal of fertilizers subsidies by most African governments has increased fertilizer prices in relation to crop prices in many of these countries (Bumb et al., 1996). Fertilizer recommendations disregard variations in crop demand and soil properties and farmers” access to inputs and commodity markets with scales that is too large to capture soil heterogeneity (Smaling et al., 2002). Farmers on their part lack of information about the best fertilizers to use for their particular fields and cropping practices, making the crop response to fertilizers more erratic and less profitable. Even within more localized recommendation domains, households operate at different stages of economic development leading to misuse and associated economic (chase et al., 1991) and environmental risks (Bundy et al., 2001).
STATEMENT OF THE PROBLEM
In Nigeria today, crop production has been largely inconsistent resulting to the lack of knowledge of the combination of soil type and fertilizer. An adequate knowledge on the right combination would help to increase crop production and maintain that for a long time. There is equally a concern by farmers and researchers on the both organic and inorganic fertilizers to be used to increase crop yield. Another problem of farmers is to know the relationship between soil type and crop yield.
Above all, this research work is highlights the response or effect of soil types and plots on crop performance, and also to find out whether fertilizer types affect the crop performance.
AIM AND OBJECTIVES OF THE STUDY
The aim of this research work is to investigate the response of crop to fertilizer types, plots and soil types. The above aim is achieved through the following objectives:
To test the homogeneity of variance among the variables (fertilizer types, plots and soil types) on crop performance.
To test for the response of fertilizer types, plots, and soil types on crop performance using multivariate tests.
To verify the variables that contribute more between soil types, plots, fertilizer type on crop performance using multivariate test.
SIGNIFICANCE OF THE STUDY
the importance of this research work is to enable both the farmers and the government to have clear view on whether effects of fertilizers and soil types really contribute to the performance of crop or not. The most importance of this study is mainly to find out if there is any relationship between fertilizer types and soil types on crop yield.
SCOPE AND LIMITATION OF THE STUDY
The scope of this study focus on crops performance to the types of soil and fertilizer used. The scope of this study is to find out whether fertilizer types, plots and soil types have impact in producing a best crop yield. There are many factors contributing the performance crops but due to the data collection, time and financial constraints, this study is limited to only knowing the response of fertilizer types, plots and soil types on the performance of crop.
DEFINITION OF SOME BASIC TERMS
Fertilizer: is the reason why plants grow. It also adds nutrients and texture to soil to provide nutrients some plants e.g. herbs, shrubs, flowers etc.
Organic fertilizer: is all natural and includes things such as bat, guano, compost, peat moss, wood ash and manure.
Inorganic fertilizer: this is primarily derived from chemical compound, either mineral or synthetic.
Crops: a plant that is grown in large quantities, especially as food.
Synthetic: this is a substances made artificial by combining chemical substances e.g. inorganic fertilizer rather than being produced by plants or animals which are the natural organisms e.g. organic fertilizer.
Soil: this is as a result of organic matter which coats soil particles, or can be define as the top layer of the earth surface, in which plants, trees etc.
CHAPTER TWO
2.0 Literature Review
2.1 Introduction
Multivariate analysis of variance (MANOVA) is simply an ANOVA with several dependent variables. This is to say, ANOVA test for the difference in means between two or more groups, while MANOVA test for the difference in two or more vectors of means. E.g. (Holloway et al.,1967).
For example, we may conduct a study where we try two different textbooks, and we are interested in the student’s improvements in statistics and physics. In that case, improvements in mathematics and physics are the two dependent variables, and our hypothesis is that both together are affected by the difference in textbooks. A multivariate analysis of variance (MANOVA) could be used to test this hypothesis. Instead of a univariate F-value, we would obtain a multivariate F-value (wilk’s ) based on a comparison of the error variance/covariance matrix and the effect variance/covariance matrix.
Although we only mention wilk’s here, there are other statistics that may be used, including hotelling’s trace and Pillai’s criterion. The “covariance” here is include because the two measures are probably correlated and we must take this correlation into account when performing the significance test.
Testing multiple dependent variables is accomplished by creating new dependent variables that maximize group differences. The artificial dependent variables are linear combinations of the measured dependent variables.
2.2 Review of Some Related Literature
In this chapter it emphases or highlight other related researches that have been made by other researchers using multivariate analysis of variance (MANOVA), to see how they come up with their ideas and conclusions, using method of multivariate.
Historically, the bulk of applications of multivariate techniques have been in the behavioral and biological science. However, interest in multivariate method has now spread to numerous other field of investigation. For example, I have collaborated on multivariate problems with researchers in education, chemistry, physics, geology, engineering, law, business, literature, religion, public broadcasting, nursing, mining, linguistics, biology, psychology, and many other fields.
Olusegun A. (2003) stated that 28% to 42% of nitrogen fertilizer applied to maize remained in the soil depending on nitrogen rate and tillage. Plassis L. (2003) found that 28% and 31% respectively, of urea and oxide nitrogen applied to sorghum were in the upper 25cm soil depth at the end of the cropping season.
Peters, et al., (1965) reported that soya beans yields increased from 1.3-1.9 t/ha when the nitrogen applications in the proceeding rice crop (residual effect) increased from 0 to 180kgN/ha. This means that the residual effect of nitrogen fertilization is affected by so many variables and is therefore site specific. The fertilizer rate applied, leaching, immobilization, DE nitrification and rainfall patterns are likely to affect the magnitude of the residual effect.
Ashalatha (1989) studies MANOVA for 17 genotypes with seven variables of Ragi at 15 locations. The results revealed that the genotype, location and GE-interactions were significant when tested against pooled error using wilk’s lambda criterion.
Ruìz-nogueira et al., (2001) field experiments were conducted on genotype x environment interaction and dry matter yield stability of white clover (Trifolium Opens l.) cultivars and breeding populations to study the dry matter yield peculiarities in 6 cultivars (Suduviai, Bitunai, Atoliai, Nemuniai, Milo and Gvendel) and 4 breeding populations (nos. 1123, 1124, 1421 and 1435) of white cover, ANOVA was employed to draw the consolations. Result of the ANOVA analysis showed that dry matter yield was essentially influenced by the cuts, year of trials, cultivars and their interactions. Promising breeding lines 1123 and 1124-combined high annual yield of dry matter with a low variance of stability (0.0593-0.0956).
Adebayo et al., (2014) field experiment was carried out at the teaching and research farm of Kwara state university, Malete, Ilorin, to evaluate the effect of compost, organ mineral, and inorganic fertilizers on the growth and yield of Amaranthus Caudatus as well as its residual effects. Amaranthus was growth with compost grade B (unlamented compost), organ mineral fertilizer Grade A (compost amended with mineral fertilizer). And NPK 15-15-15 and no fertilizer (control). All the treatments except control were applied at the rate of 100kgN/ha. The results indicated that Amaranthus yield of 18.9t/ha produced from grade A was significantly (P<0.05) higher than 17.6t/ha obtained from NPK fertilizer. Residual effect of Amaranthus growth parameters such as plant height , number of leaves, and yield values obtained from grade A was also significantly (P<0.05) higher than that of NPK, compost, and control values. Thus, organ mineral fertilizer could be used in cultivation of Amaranthus Caudatus in Ilorin and in similar type of soil in similar agro ecology.
Rachel et al., (2016) lonicera Maackii is an invasive Asian shrub naturalized in North America that has negative effects on forest understory vegetation. Aqueous extracts of leaves and roots of this plant have been shown to inhibit germination of several species. He examined the extent to which field-collected soils conditioned by L. Maackii growth, with and without additions of L. Maackii extracts, had effects on growth, morphology, and reproduction of Arabidopsis thaliana in a greenhouse setting. We also examine the effect of nutrient addition to the same soils on the performance of A. thaliana, whether plant responses to nutrients varied among L. Maackii- conditioned and unconditioned soils, and whether the effects of L. Maackii extracts varied in soils with different histories and fertility. Plant grown in forest soil collected from a site where L. Maackii was present grew slower and flowered later than plants grown in an unconditioned soil, but ended up being larger, with more leaves, branches and a higher total seed output. Nutrient addition had a positive effect on performance of A. thaliana, but when nutrients were added with extracts of L. Maackii roots and leaves, the positive effects of nutrient were greatly diminished. Inhibiting plant response to resource opportunities is a potentially important indirect effect of all alchemical. When direct effects of extracts on growth were seen, leaf extracts had a greater effect than root extracts. While the most important impacts of L.maackii in the field are undoubtedly due to competition for light and moisture, evidence for all allopathic effects of this plant is growing.
Zublena et al., (1990) earlier observed that poultry manure in crop husbandry must be done with sound soil fertility management practices to prevent soil nutrient imbalance and associated animal and human health risks, as well as surface-water and groundwater contamination. Therefore, addition of poultry manures soil for enhanced fertility must be done with caution.
Makind et al., (2010) earlier obtained similar increase in plant height of A. cruentus, though with increased organ mineral fertilizer applications in orthic luvisol and Dystric fluvisol soils gave similar results of increased leafy productivity of grain amaranth when the combination of poultry manure and urea-N fertilization was compared with conventional fertilizer application.
Alley and Vanlauwe (2009) defined soil fertility as the capacity of soil to retain, cycle and supply essential nutrients for plant growth over extended periods of time. It is important to crop production as the soil is the nutrient base for plants but low soil fertility, particularly N and P deficiencies, is one of the major biophysical constraints affecting African agriculture (Mokwunye et al., 1996). The import of these is that no matter how other factors of production are remedied, food production in Africa will continue to decrease unless there is conscious effort to address soil fertility depletion. Nutrient depletion rates are field specific, depending on the way each particular field has been managed over decades (Sanchez et al., 1997) hence the replenishment should also be field/site specific for effective results. Nutrient depletion rates vary with soil properties. The proportion of nutrient loss is normally greater in sandy soils because soil organic matter (SOM) particles are less protected from microbial decomposition in sandy soils than in loamy or clayey soils (swift et al., 1994).
Burow KR et al., (2003). In his study, he examined the influence of different nitrogen (N) application rates (0, 168, 240, 270 and 312 kg N ha-1) on soil properties, maize (Zea Mays L.) yield and microbial communities of three types of soils (clay, alluvial and sandy soils). Results indicated that N fertilization significantly decreased microbial biomass in both clay and sandy soils regardless of application rate. These decreased were more likely a result of soil pH decreases induced by N fertilization, especially in the sandy soils. This is supported by structural equation modeling and redundancy analysis results. Nitrogen fertilization also led to significant changes in soil microbial community composition. However, the change differences were gradually dismissed with increase in N application rate. We also observed that N fertilization increased maize yields to the same level regardless of application rate. This suggests that farmers could apply N fertilizers at a low rate (i.e. 168 kg N ha-1), which could achieve high maize yield on one hand while maintain soil microbial functions on the other hand.
Emmanuel (2014). The attendant low yield of cowpea among smallholder farmers has increased the need for site specific fertilizer recommendation and integration of biological materials to increase the yield of crop. The effectiveness of applied fertilizer is constrained by the use of the inappropriate rate and improper timing of sowing. Rhizobia Inoculant, on the other hand, needs a balanced nutrient application to increase crop yield. The study was designed to: i) determine the effect of inoculants, P and K mineral fertilizers on N, P, K uptake, growth and grain yield of cowpea, ii) evaluate the effect of NPK fertilizer application on the growth and yield of cowpea in the Guinea and Sudan savanna zones of Ghana, and iii) simulate the potential yield, yield gap, best sowing date, growth and yield of cowpea using CROPGRO cowpea DSSAT model. The response of cowpea to Rhizobia inoculation and fertilizer application revealed that sole application of inoculants was not sufficient to raise cowpea yield except when combined with 30 kg P205 ha-1 and 20 kg K20 ha-1. The yield response to mineral fertilizer also showed that K is very essential in determining cowpea yield and should therefore, not be omitted in any fertilizer recommendation rate. CROPGRO – cowpea model was used to simulate the response of cowpea to different N rates, potential yield and the best sowing dates for cowpea. The model was calibrated for Omondaw cowpea cultivar using data from the experiment carried out at Lawra (ferric Lixisol) and Nyoli (ferric Luvisol) during the 2012 and 2013 cropping seasons. The model performance was evaluated statistically using RMSE (0.13 tons ha-1), CV (RMSE) (9.9%) and Wilmott index of agreement d (0.97). A long term seasonal analysis using the model was able to detect that 15kg N ha-1 is optimal for ferric Lixisol while 20kg N ha-1 was optimal for ferric Luvisol.to avoid crop failure, sowing window for Lawra range from july 11 xvii to july 21st while that of Nyoli was extended to 26th July with consideration for august 5th. The yield gap analysis revealed that a wide gap exists between climatic potential yields and yields obtained from farmers’ fields. The gaps between potential and research station yields (yield gap 1) range from 1.05 to 18% of potential yield, while that of the farmer’s field was 84.21% for Lawra soil and 79.55% for Nyoli soil. It is necessary to reduce the yield gap by using site specific fertilizer recommendation and appropriate timing of sowing dates.
CHAPTER THREE
3.0 Methodology
3.1 Introduction
This chapter consists of method of data collection used for this study and method of data analysis. Method of data collection section gives details on how the data were collected and method of data analysis, and also in this section the explanation of the statistical tools and statistical software used for the analysis.
3.2 Research Design
This study focused on an investigation of what cause the other among different variables. This study is most preferred because the study will be investigation the response of crop to fertilizers and soil types. This study adopted both descriptive and explanatory research design. First, the study described the crop performance on soil and fertilizer types.
3.3 Data Description
In the course of this study, the data used for the analysis was strictly the secondary data. It was extracted from the research and record book of Najanu Memorial Farms Kaura Namoda Zamfara state.
3.4 Multivariate Variables Specification
The multivariate variables model for the study consists of three constructs as described below:
soil type
fertilizer type used
crop performance
Soil Type
Firstly, soil is as a result of organic matter which coats soil particles, Brady, N.C. (2000). The soil type used for this study consists of the following three types of soil:
loamy soil type
sandy soil type
clay soil type
Loamy Soil Type
Loamy soil is the most desirable soil texture for most plant cultivation. The loam class of soil presents the optimum habitat for plant health. It retains moisture without impeding drainage, captures nutrients and allows oxygen and microorganisms to circulate. There are different of loam soil, classified according to the composition of the soil particles contained in a sample. Jenny, Hans. (1999).
Sandy Soil
This is granular soil that contains small rock and mineral particles. The texture of sandy soil is usually gritty. This soil feel course whether it is dry or wet. Sandy soils are the result of the weathering and disintegration of a variety of rocks such as granite, limestone and quartz. This type of soil is easy to cultivate but, since it allows for more drainage than needed, it is important to water it regularly, especially during summer days. As sandy soil doesn’t allow the water to pool around the roots, they are a good choice for plants that have a tendency to suffer from root decay. Millar et al., (2002).
Clay Soil
This is a fine-grained natural rock or soil materials that combine one or more clay minerals with traces of metal oxides and organic matter. Clays are plastic due to their water content and become hard, brittle and non-plastic upon drying or firing. (Wikipedia the free encyclopedia, 2015)
Fertilizer Types
Fertilizer is an essential part of growing plants. It adds nutrients and texture to soil to provide nutrient to trees, vegetables, herbs, shrubs and flowers. There are several ways to categorize fertilizer, and the most basic is whether it is organic or inorganic.
Organic fertilizer
Inorganic fertilizer
Organic Fertilizer
Is all natural and includes things such as bat guano, compost, peat moss, wood ash and manure. Singer, et al.,(2000)
Inorganic Fertilizer
This is primarily derived from chemical compounds, either mineral or synthetic. They typically come as a powder, pellets, granules or a liquid. Most inorganic, concentrated fertilizer is rated based on the percentage of nitrogen, phosphorous and potassium. Other chemicals that might be included in inorganic fertilizers include calcium, sulfur, iron, zinc magnesium.
Crop Performance
The performance of crops was rated on a scale of 1 to 5. With 5 signifying crops that performed very well and 1 signifying poor performance of crops.
3.5 Statistical Methodology
The data to be analyzed consist of independent variables represented by the treatments accordingly: i.e. plots, soil types and fertilizers used (inorganic and organic) and the dependent variable i.e. the crop performance. We wish to observe the effects of treatment on the independent variables simultaneously.
MANOVA Advantages over ANOVA
It tests the effects of several independent variables and outcome (dependent) variables within a single analysis
Independent variables of interest are likely to affect a number of different conceptual variables - for example: in the case of this research topic.
Since only ‘one’ dependent variables is tested, the researcher is protected against inflating the type 1 error due to multiple comparisons.
Assumption Of Multivariate Analysis Of Variance (MANOVA)
In multivariate analysis of variance (MANOVA) the following assumptions are made when using a MANOVA. Wilks, S.(1946)
Normal Distribution
The dependent variable should be normally distributed within groups. Overall, the F test is robust to non-normality, if the non-normality is caused by skewness rather than by outliers.
Linearity
MANOVA assumes that there are linear relationships among all pairs of dependent variables in each cell. Therefore, when the relationship deviates from linearity, the power of the analysis will be compromised.
Homogeneity of Variances
Homogeneity of variances assumes that the dependent variables exhibit equal levels of variance across the range of predictor variables. Remember that the error variance is compute (Ss error) by adding up the sum of squares within each group. If the variances in the two groups are different from each other, then adding the two together is not appropriate, and will not yield an estimate of the common within-group variance.
Homogeneity of Variances and Covariances
In multivariate designs, with multiple dependent measures, the homogeneity of variance assumption described earlier also applies. There are various specific tests for this assumption has minimal impact if the groups are of approximately equal size. Therefore, every effort should be made to have equal cell sizes. (Alin and Kurt, 2006),
Significance Test of Multivariate Analysis of Variance (MANOVA)
In statistics, multivariate analysis of variance (MANOVA) is a procedure for comparing multivariate sample means. As a multivariate procedure, it is used when there are two or more dependent variables, and is typically followed by significance test involving individual dependent variables separately. It helps to answer so many questions one of which is:-
Do changes in the independent variable(s) have significant effects on the dependent variables?
3.6 Hypothesis Testing In MANOVA
The reason for four different statistics and for approximations is that the mathematics of MANOVA get so complicated in some cases that no one has ever been able to solve them. To understand MANOVA, it is not necessary to understand the derivation of the statistics. Here, all that is mentioned is their names and some properties.
The four most widely used measures for assessing significance between groups on the independent variables are:
1. Pillai’s Trace
Hotelling- lawley Trace
Wilk’s Lambda (
Roy’s Largest Root
3.6.1Pillai’s Trace
The Pillai’s-Bartlett trace is the sum of explained variances on the discriminant variates , which are the variables which are computed based on the canonical coefficients for a given root. The larger the Pillai’s trace, the more the given effect contributes to the model. Pillai’s trace is always smaller than Hotelling’s trace. This is the most robust test of all each measure/test is preferred in differing situations. Pillai, K.C.S. (1964)
Pillai’s criterion is considered more robust and should be used if sample size decreases, unequal cell sizes appear or homogeneity of covariance is violated. Some statisticians consider it to be the most powerful and most robust (type I sense) of the four statistics. An alternative criterion for testing the null hypothesis H0: = … , was proposed by Pillai’ K.C.S et al., (1965). The formula for the Pillai’s trace is given as:-
Pillai’s trace: V2 = (3.1)
Hotelling- Lawley’s Trace
It is a test to assess the statistical significance on the difference of the means of two or more variables between the groups. It is special case of MANOVA used with two groups of a treatment variable. The larger the Hotelling’s trace, the more the given effect contributes to the mode. Hotelling, H. (1951) is defined as
Hotelling’s trace: T = (3.2)
Wilk’s Lamda (
Wilk’s lambda is a test statistics used in multivariate analysis of variance (MANOVA) to test whether there are differences between the means of identified groups of subjects on a combination of dependent variables. For example, we have to test whether the mean score of two groups, graduates and diplomats, is the same across eight constructs simultaneously. Thus, there are eight dependent variables and comparing the mean of this combination for two groups. Wilk’s lambda performs, in the multivariate setting with a combination of dependent variables, the same role as f-test performs, in one-way analysis of variance. Wilk’s lambda is a direct measure of the proportion of variance in the combination of dependent variables that is unaccounted for by the independent variable (the grouping variable or factor). If a large proportion of the variance accounted for by the independent variable then it suggests that there is an effect from the grouping variable and that the groups (in this case the graduates and diplomats) have different mean values. The t-test, Hotelling’s T, and F-test are special cases of wilk’s lamda. Wilk’s lamda ranges from 0 to 1 and the lower the wilk’s lamda, the more the given effect contributes to the model.
Wilk’s was the first manova test statistic developed and is very important for several multivariate procedures in addition to manova. It was proposed by s.s wilk’s (1906-1964). This statisticwilk’s (1932), which is often called the wilk’s which may be defined as the ratios of the two determinants (variance) of S matrices are found. Wilk’s the test statistic preferred for MANOVA, and is found through a ratio of the determinants
Mathematically as:
(3.3)
With e
The following approximation based on the f-distribution is used to determine significance levels.
We reject H if Note that the rejection is for small values of Wilk’s. The parameters in wilk’s
p= number of variables (dimension)
h= degree of freedom for hypothesis
e= degree of freedom for error.
The quantity (1- is often interpreted as the proportion of variance in the dependent variables explained the model effect. However, this quantity is not unbiased and can be quite misleading in small samples.
Determination involves finding functions of matrix called eigenvalues. The matrix of interest for the Wilk’s is actually product of two matrices E-1H, where the matrices, E and H, are as described earlier. The eigenvalues are obtained by solving the determinant equation -1H – Thus, wilk’s
Wilk’s lambda: ) (3.4)
The number of nonzero eigenvalues of E-1H is q = min (p,h) which is the rank of the H. The matrix HE-1 has the same eigenvalues as HE-1 and could be used in its place to obtain however we prefer HE-1 because we use it in eigenvectors.
Roy’s Largest Root
This test measures the differences on only the first discriminant function among the dependent variables. This criterion provides advantages in power and specificity of the test but makes it less useful in certain situations where all dimensions should be considered. This test is more appropriate when dependent variables are strongly interrelated on a single dimension, but it is also the measure most be severely affected by violations of the assumptions. This is often test for H0: = = …. = based on we use Roy’s union-intersection the test statistic is computed as:
Roy’s largest root: (3.5)
The maximum eigenvalue of A-1 (Recall that a “root” is another name for an eigenvalues) Hence, this statistic could also be called Roy’s largest eigenvalue. And eigenvectors of -1
In some cases, the four will generate identical F statistics and identical probabilities. In other’s they will differ. When they differ, Pillai’s trace is often used because it is the most powerful and robust (type I sense). Because Roy’s largest root is an upper bound on f, it will gives lower bound estimate of the others are not significant.
Generally, if group sizes are equal, the tests are sufficiently robust with respect to heterogeneity of covariance matrices so that we need not worry. If the ni’s are unequal and we have heterogeneity, then the -level of the manova test may be affected as follows. If the larger variance and covariance are associated with the larger samples, the true -level is reduced and the result becomes conservative. On the other hand, if the larger variance and covariance come from smaller samples, is inflated, and the tests become liberal.
Statistical Package To Be Used For The Study
MANOVA analysis can be conducted by using several statistical package or soft wares but in the case of this research work or project MINITAB is the statistical software to be used for analysis of this research work. And also for homogeneity of variance R-package was used to see if the homogeneity among the variance exists.
Data Description
The data collected on the crop performance base on the soil types and different types of fertilizer being into two different types of plots, in which organic fertilizer is applied to plot one and inorganic fertilizer is applied to plot two
Plots
Soil types
Fertilizer types
Crop performance
Plot1
Plot2
Loamy
Sandy
Clay
Organic
Inorganic
Very Poor
Poor
Fair
Well
Very Well
1
2
1
2
3
1
2
1
2
3
4
5
Data Analysis
Table 3.9.1: Bartlett Test of Homogeneity of Variance
Test
DF
p-value
Bartlett’s
33.055
3
3.135e-07
From the above table the p-value (3.135e-07) is less than the value of alpha (0.05), we therefore reject the null hypothesis and conclude that the homogeneity among the variance exist.
Table 3.9.2: MANOVA for plots
Criterion
Test Statistic
F
DF
P
Wilk’s
0.89888
0.900
(1, 8)
0.371
Lawley-Hotelling
0.11250
0.900
(1, 8)
0.371
Pillai’s
0.10112
0.900
(1, 8)
0.371
Roy’s
0.11250
From the table 3.9.2.Above, we have the following conclusions;
Decision: the p-value (0.371) corresponding to the plots is greater than the value of alpha (0.05). Therefore, we accept the null hypothesis
Wilk’s lambda ( test: from this test we concluded that plots factor has significant effect on crop performance at 5% level of significance.
Lawley-Hotelling test: this test testified us that the means between the crop performance and plots does not differ significantly i.e. is equal at 5% level of significance.
Pillai’s test: this test shows us that the relationship that exists between the crop performance and plots is significantly least at 5% level of significance.
Roy’s test: it testified that plots factor has no significant effect on crop performance when the remaining independent variables (fertilizer and soil types) were not considered at 5% level of significance.
Table 3.9.3: MANOVA for soil types
Criterion
Test Statistic
F
DF
P
Wilk’s
0.84325
0.744
(2, 8)
0.506
Lawley-Hotelling
0.18588
0.744
(2, 8)
0.506
Pillai’s
0.15675
0.744
(2, 8)
0.506
Roy’s
0.18588
From the table 3.9.3 above, we have the following conclusions;
Decision: the p-value (0.506) corresponding to the soil types is greater than the value of alpha (0.05). Therefore, we accept the null hypothesis
Wilk’s lambda ( test: from this test we deduced that the soil types have no significant effect on crop performance at 5% of significance.
Lawley-Hotelling test: this test testified us that the means between the crop performance and soil types does not differ significantly i.e. is equal at 5% level of significance.
Pillai’s test: this test shows us that the relationship that exists between the crop performance and soil types is significantly least at 5% level of significance.
Roy’s test: it testified that soil types have no significant effect on crop performance when the remaining independents variables (fertilizer and plots) were not considered at 5% level of significance.
Table 3.9.4: MANOVA for fertilizer types
Criterion
Test Statistic
F
DF
P
Wilk’s
0.11348
62.500
(1, 8)
0.000
Lawley-Hotelling
7.81250
62.500
(1, 8)
0.000
Pillai’s
0.88652
62.500
(1, 8)
0.000
Roy’s
7.81250
From the table 3.9.4 above, we have the following conclusions;
Decision: the p-value (0.000) corresponding to the fertilizer types is less than the value of alpha (0.05). Therefore, we reject the null hypothesis
Wilk’s lambda ( test: from this test we observed that the fertilizer types have significant effect on crop performance at 5% level of significance.
Lawley-Hotelling test: this test testified us that means between the crop performance and fertilizer types does differ significantly i.e. is not equal at 5% level of significance.
Roy’s test: it testified that fertilizer types have significant effect on crop performance when the remaining independents variables (soil types and plots) were not considered at 5% level of significance.
Table 3.9.5: MANOVA for interaction between plots and soil types
Criterion
Test Statistic
F
DF
P
Wilk’s
0.66824
1.986
(2, 8)
0.199
Lawley-Hotelling
0.49647
1.986
(2, 8)
0.199
Pillai’s
0.33176
1.986
(2, 8)
0.199
Roy’s
0.49647
From the table 3.9.5 above, we have the following conclusions;
Decision: the p-value (0.199) corresponding to the interaction of plots and soil types is greater than the value of alpha (0.05). Therefore, we accept the null hypothesis
Wlik’s lambda test: from this test we concluded that the interaction of plots and soil types has no significant effect on crop performance at 5% level of significance.
Lawley-hotelling test: this test testified us that the means between the crop performance and the interaction of plots and soil types does not differ significantly i.e. is equal at 5% level of significance.
Pillai’s test: this test shows us that the relationship that exists between the crop performance and the interaction of plots and soil types is significantly least at 5% level of significance.
Roy’s test: it testified that the interaction of plots and soil types have no significant effect on crop performance when the remaining independent variable (fertilizer) was not considered at 5% level of significance.
Table 3.9.6: MANOVA for interaction between plots and fertilizer types
Criterion
Test Statistic
F
DF
P
Wilk’s
0.62016
4.900
(1, 8)
0.058
Lawley-Hotelling
0.61250
4.900
(1, 8)
0.058
Pillai’s
0.37984
4.900
(1, 8)
0.058
Roy’s
0.61250
From the table 3.9.6 above, we have the following conclusions;
Decision: the p-value (0.058) corresponding to the interaction of fertilizer types and plots is less than the value of alpha (0.1). Therefore, we reject the null hypothesis
Wlik’s lambda ( test: from this test we observed that the interaction of fertilizer types and plots has significant effect on crop performance at 10% level of significance.
Lawley-Hotelling test: this test testified us that the means between the crop performance and that of interaction between fertilizer types and plots does differ significantly i.e. is not equal at 10% level of significance.
Pillai’s test: this test shows us that the relationship that exists between the crop performance and the interaction of plots and fertilizer types is significantly high at 10% level of significance.
Roy’s test: it testified that the interaction of plots and fertilizer types has significant effect on crop performance when the remaining independent variable (soil types) was not considered at 10% level of significance.
Table 3.9.7: MANOVA for interaction between soil types and fertilizer types
Criterion
Test Statistic
F
DF
P
Wilk’s
0.52861
3.567
(2, 8)
0.078
Lawley-Hotelling
0.89176
3.567
(2, 8)
0.078
Pillai’s
0.47139
3.567
(2, 8)
0.078
Roy’s
0.89176
From the table 3.9.7 above, we have the following conclusions;
Decision: the p-value (0.078) corresponding to the interaction of fertilizer types and soil types is less than the value of alpha (0.1). Therefore, we reject the null hypothesis
Wlik’s lambda ( test: from this test we observe that the interaction of fertilizer types and soil types has significant effect on crop performance at 10% level of significance.
Lawley-Hotelling test: this test testified us that the means between the crop performance and that of interaction between fertilizer types and soil types does differ significantly i.e. is not equal at 10% level of significance.
Pillai’s test: this test shows us that the relationship that exists between the crop performance and the interaction of fertilizer types and soil types is significantly high at 10% level of significance.
Roy’s test: it testified that interaction of soil types and fertilizer types has significant effect on crop performance when the remaining independent variable (plots) was not considered at 10% level of significance.
Table 3.9.8: MANOVA for interaction between plots, soil types, and fertilizer types
Criterion
Test Statistic
F
DF
P
Wilk’s
0.55921
3.153
(2, 8)
0.098
Lawley-Hotelling
0.78824
3.153
(2, 8)
0.098
Pillai’s
0.44079
3.153
(2, 8)
0.098
Roy’s
0.78824
From the table 3.9.8 above, we have the following conclusions;
Decision: the p-value (0.098) corresponding to the interaction of three independent factors is less than the value of alpha (0.1). Therefore, we reject the null hypothesis
Wlik’s lambda ( test: from this test we observed that the interaction of three independent factors has significant effect on crop performance at 10% level of significance.
Lawley-Hotelling test: this test testified us that the means between the crop performance and that of interaction between the three independent factors does differ significantly i.e. is not equal at 10% level of significance.
Pillai’s test: this test shows us the relationship that exists between the crop performance and the interaction of three independent factors is significantly high at 10% level of significance.
Roy’s test: it testified that the interaction of soil types, plots and fertilizer types have significant effect on crop performance at 10% level of significance.
Table 3.9.9: General ANOVA for crop performance versus plots, soil, and fertilizer
Source
DF
S.S
MS
F
P
Plots
1
5.8814
5.8814
4.0044
0.371
Soil types
2
4.0512
2.0256
1.37913
0.506
Fertilizer types
1
38.8687
38.8687
26.4638
0.000
Plots and soil types
2
0.2325
0.11625
0.07914
0.199
Plots and fertilizer types
1
0.9259
0.9259
0.6304
0.058
Soil types and fertilizer types
2
5.0000
2.5000
1.70213
0.078
Plots, soil types, and fertilizer types
2
0.0000
0.0000
0.0000
0.098
Error
8
11.7500
1.46875
Total
19
66.7097
The table 3.9.9 above shows the analysis of variance for the crop performance versus plots, soil types and fertilizer types. Hence the result confirmed the earlier discussion above that fertilizer types and any interaction with fertilizer types has significant effect on the crop performance, there by rejecting the null hypothesis at specified level of significance, whereas the remaining factors and interaction has no significant effect on the crop performance. Thus, this signifies that fertilizer types have a strong impact on the performance of crops.
CHAPTER FOUR
4.0 SUMMARY, CONCLUSION AND RECOMMENDATION
4.1 Summary
The project work titled multivariate analysis of variance (MANOVA) on the response of crop performance to fertilizer types, plots and soil types. This project reflects on how it has met its target aims and objectives, stated its statement of the research problem, significance of the study, scope and limitation.
Related literature were reviewed, source of case study, method of data collection, method of data analysis which use the MINITAB and R-package for the analysis. The analysis which included test of homogeneity of variance using Bartlett’s test, conclusion and recommendation were made
4.2 Conclusions
From the view of the above chapter three interpretations of results the following conclusions can be observed:
The result obtained from the Bartlett’s test of homogeneity or equality of variance shows that there is equality of dependent variable across the group at 5% level of significance. And from the multivariate analysis result we observed that the fertilizer types and any interaction with fertilizer types has significant effect on the crop performance, there by rejecting the null hypothesis at specified level of significance, whereas the remaining factors and interaction has no significant effect on the crop performance. Thus, this signifies that fertilizer types have a strong impact on the performance of crop; this was even justified from the ANOVA result.
4.3 Recommendations
1. Future researchers should carry out similar research work with more than three interaction effect to compare the results.
2. It is recommended if the future research focused on finding out what fertilizer type is suit soil best.
3. Moreover, the future research can also find out the best combination between soil types and specific crop.
4. It is advisably to farmer to adopt using both fertilizer types more especially applying organic first before inorganic fertilizer because organic helps inorganic in given a better yield.
REFERENCES
Alin and Kurt S. (2006). Statistical methods in medical research”.
Alley, M.M. and Vanlauwe, B. (2009). “The role of fertilizers in integrated plant nutrient
management First edition”, IFA, Paris, France, TSBF-CIAT, Nairobi Kenya.
Anaike, O.E. (2008). A project work on two-way MANOVA with interaction in physical
science of imo state university, Owerri, Department of sciences. Unpublished B.Sc project.
Ashalatha KV., (1989). A statistical Appraisal of Genotype-Environment interactions in Ragi
(Eleusine Coracana, G) under multicocation trails. M.Sc (agric), thesis university of Agricultural Sciences, Bangalore (India).
Bontkes, T.E.S and Wopereis, M.C.S (2003). Perspectives for the use of decision support tools
in agricultural research and development in Sub-Sahara Africa, pp. 150-158. in T.E Struif Bontkes and Wopereis, M.Sc (eds.) decision support tools for smallholder agriculture in sub-Sahara Africa a practical guide. IFDC and CTA.
Brady, N.C (2000). The nature and properties of soil 10th Ed. New York: Mcmillan.
Bumb, B.l.and Baanante, C.A. (1996). The role of fertilizers in sustaining food security and
protecting the environment to 2020. Food, Agric. And the Environ. Dis. Pap. 17. Int. food policy res. Inst., Washinton, DC.
Bundy, l.G., Andraski, T.W. and Powell, J.M. (2001). Management effects on phosphorus
losses in runoff in corn production systems. Journal of environmental quality, 30: 1822-1828. 131.
Burow KR, Nolan BT, Rupert MG, Dubrovsky NM. (1991-2003) nitrate in groundwater of
the united states,. Environ sci technol. 2010; 44: 4988-4997. DOI: 10.1021/es100546y [Pubmed].
Camara O, and Heinemann, E.D. (2006). overview of fertilizer situation in africa, 27 pp. in
proceedings of the African fertilizer summit. June 9-13, 2006, Abuja , Nigeria.
Chase, C., Duffy, M., Webb, J. and Vosss, R.(1991). An economic assessment of maintaining
High phosphorus and potassium soil test levels. American journal of alternative Agriculture 6: 83-86.
Emmanuel Obianuju Chiamaka. (2014) “a thesis presented to the department of crop and soil
sciences, faculty of agriculture, college of agriculture and natural resources, kwame Nkrumah university of science and technology, Kumasi Ghana, in partial fulfillment of the requirements for the award of degree of doctor of philosophy in soil sciences”.
Everitt B.S & Dunn G. (1991) applied multivariate data analysis. Edward Arnold. London.pp. 219-220.
Gerner, H., Asante, E.O., Owusu Bennoah, E. and Marfo, K.(1995). Ghana fertilizer
privatization scheme. Private sector role and public sector responsibilities in meeting needs of farmers. IFDC- Africa, Lome Togo.
Heisey, P.W. and Mwangi, W. (1996). Fertilizer use and maize production in sub-Sahara
Africa. Econ. Work. Pap. 96-01. CIMMYT, Mexico City.
Holloway, l.N. and Dunn, O.J (1967) the robustness of Hotelling T2. Journal of the America
statistical association, 62, 124-136.
Hotelling, H. (1951), “the generalization of student’s ratio,” annals of mathematical statistics, 2, 360-378
Jenny, Hans. (1999). Factors of soil formation; a system of quantitative pedology. (originally
published by mcgraw-hill; mineola, n.y.dover).
Kan’ankuk’a, C.N. (1999). Effect of lime, n and p on growth, yield and yield components of
cowpea (v.unguiculata [l] walp.). thesis submitted to the postgraduate school, Ahmadu Bello University, Zaria, 110 pp.
Makinde, E.A., l.S., Ojeniyi, S.O. 2010. Morphological characteristic of Amaranthus cruentus l. as influenced by kola pod husk, organomineral and NPK fertilizers in southwestern Nigeria. New York science journal 3(5): 130-134.
Millar, C.E., and Turk, l.M. (2002). Fundamentals of soil science. New Delhi: Biotech Books.
Mokwunye, A.U., de Jager, A. and Smaling, E.M.A. (1996). Restoring and maintaining the
productivity of West African soils: key to sustainable development. Misc fert. Stud. 14. International fertilizer development centre (IFDC)- Africa , Lome, Togo.
OLowoake Adebayo Abayomi and Ojo James Adebayo (2014) development of crop
production, Kwara State University, PMB 1530, Ilorin, Nigeria correspondence should be addressed to Olowoake Adebayo Abayomi; aolowoake@yahoo.com received 10 September 2014; revised 9 November 2014; accepted 10 November 2014; published 20 November 2014 academic editor: albino Maggio copyright © 2014 o. Adebayo Abayomi and O. James Adeb
Pillai, K.C.S. (1964), “on the distribution of the largest of seven roots of a matrix in multivariate
analysis,” biometrika, 51,270-275.
Pillai, K.C.S. (1965), “On the distribution of the largest characteristic root of a matrix in
multivariate analysis,” Biometrika, 52, 405-414.
Rachel E. Mcneishand Ryan W. Mcewan. (2016) a review on the invasion ecology of amur
honeysuckle (Loniceramaackii, Caprifoliaceae) a case study of ecology impacts at multiple scales. The journal of the Torrey botanical society 143:4, 367-385. Online publication date: 1-aug-2016.
Ruiz- Nogueira, B., Boote, K.J. and SAU, F (2001). Calibration and use of cropgro-soybean
model for improving soybean management under Rain fed conditions. Agricultural system 68(2): 151-173
Sanchez, P.A and Leakey, R.R.B., (1997). Land use transformation in Africa: three
determinants for balancing food security with natural resource utilization. European journal of agronomy 7:1-9.
Sanchez, P.A., Buresh, R.J and Leakey, R.R.B. (1997). Trees, soils, and food security
philosophical transactions of the royal society London, biological sciences 352 (1356): 949-961.
Sangaré M.I., Ladrette C.,Mungroop RR and Berthé A. 1988. Contraintes et ameliorations
de la traction animale en mali-sud:l’experience de la DRSPR pp. 191-211 in: P.H. Starkey and F. Ndiamé (eds), animal power in farming systems. Proceedings of network shop held 17-26 September 1986 in Freetown, Sierra Leone. Vieweg for German appropriate technology exchange, GTZ, Eschborn, Federal Republic of Germany. 363p. (e/f).
Sanginga, N. and Woomer, P.l. (2009). Integrated soil fertility management in Africa:
principles practices and development process. Tropical soil biology and fertility institute of the international centre for tropical agriculture. Nairobi. 263, pp.
Singer, M.J., and Munns, D.N. (2000). Soils: an introduction. USA: prentice-hall, Inc.
Smaling. E.M.A., stoorvogel, J.J., de Jager, A. (2002). Decision making on integrated nutrient
management through the eyes of scientist, the land user and the policy maker, pp. 265-284. In Vanlauwe, B., Diels, J., Sanginga, N. and Merckx, R. (Eds.), integrated plant nutrient management in sub-Sahara Africa. from concepts to practice. CAB international, Wallingford, Oxon, UK.
Starkey P.H 1988d.animal traction research in southern mali. Consultancy report for division de recherché Surles Systemses de Production Rurale (DRSPR), Sikasso, Mali. 30p. (e,f). (Unpublished).
Swift, M.J., Seward, P.D., Frost, P.G.H., Qureshi, J.N. and Muchena, F.N. (1994). Long-
term experiments in developing a database for sustainable land use under global change, pp. 229-251. In R.A. Leing and A.E. Johnson (eds.) long-term experiments in agricultural and agricultural and ecological sciences. Cab int., Wallingford, England.
Wall, F.J. (1967), “the generalize variance ratio or u-statistic”, Albuquerque, N.M.
White S., 1985. The Bauchi State Agricultural Development Project. Draught animal news
(centre of tropical veterinary medicine, Edinburgh) no. 4:17-20. (E).
Wilks, S.S. (1932), “certain generalizations in the analysis of variance”, Biometrika, 24, 471-
494.
Wilks, S.S (1946), “sample criteria for testing equality of means, equality of variances and
equality of covariances in a normal multivariate distribution”, annals of mathematical statistics, 17, 257-281.
Wilks, S.S. (1963), “multivariate statistical outliers”, Sankhya, series A, 25, 407-426.
Zingore, S., Murwira, H.K., Delve, R.J. and Giller, K.E. (2008). Variable grain legume
yields, responses to phosphorus and rotational effects on maize across soil fertility gradients on African smallholder farms. Nutr cycl agroecosyst 80: 1-18.
Zublena, J.P., Barker J.C., Carter T.A. 1990. Soil facts: poultry manure as a fertilizer source.
North Carolina extension services, publication A G-439-5.
(A CASE STUDY OF NAJANU MEMORIAL FARMS KAURA NAMODA ZAMFARA STATE)
BY
USMAN ABDULMALIK
ADM NO: 1310212018
A PROJECT RESESARCH SUBMITTED TO THE DEPARTMENT OF MATHEMATICS, STATISTICS UNIT IN PARTIAL FULFILMENT OF THE REQUIREMENT FOR THE AWARD OF BACHELOR OF SCIENCE (B.Sc) DEGREE IN STATISTICS KEBBI STATE UNIVERSITY OF SCIENCE AND TECHNOLOGY, ALEIRO.
OCTOBER, 2017
CERTIFICATION
This is to certify that this project work was carried out by USMAN ABDULMALIK with Admission number 1310212018 in the department of mathematics, statistics unit is fully adequate in scope and qualify for the award of Bachelor of Science (B.Sc) degree in statistics Kebbi state university of science and technology, Aliero. (KSUSTA).
Dr. Onwuka Gerald Ike Date
Project Supervisor
Dr. R. V. K. Singh Date
Head of Department
Prof R. A. Apinyomi Date
External Examiner
DEDICATION
This research project is dedicated first to the “Almighty Allah” for his enabling strength he bestowed on me in completing this work.
Secondly to my late brother Jamaluddeen Usman.
ACKNOWLEDGEMENT
First of all, I want to thank Allah for his mercy on me and the strength of reaching this stage of my study. And also my sincere gratitude goes to my supervisor, Dr Gerald .I Onwuka for his supervision, assistance and piece of advice throughout the compilation of this work. I pray Allah to recompense him abundantly.
I would like to extend my gratitude profoundly to all my lectures for the knowledge impacted on me that made this enviable feat, among which are Dr. Gerald .I Onwuka, Dr. R. V. K Singh, Dr. Babayemi A. W., Dr. Nweze, Mrs. T. O. James and all the lectures of the department I pray to Allah (S.W.T) to reward them all immensely.
My deepest love and gratitude goes to my father, ALH. Usman B. Umar kwasara for his encouragement, prayers and financial support through the course of this feat. I pray to Allah (S.W.T) to bless him with much more fruitful years in life and then crown him with Aljannat Firdausi in hereafter (Ameen). It is in this view that I most extend my profound and diligent gratitude to my mother HAJ. Khadija usman kwasara I pray to Allah (S.W.T) reward her immensely in the hereafter and may He Allah increase her in good deed and fruitful years (Ameen). And I equally appreciate the wonderful contribution of my beloved step mother HAJ. Hauwa`u Usman kwasara for her support and advice I pray may Allah (S.W.T) to always replenish them purse externally and make her also an inmate of Aljannat in hereafter (Amen). These dissertation would be incomplete if I failed to recognized my beloved brothers and sisters for your love and care may Allah continue to guide you until you reach beyond where I stand today (Ameen). And also my sincere gratitude to my friends and colleagues, among which are Emmanuel sule, Bashar Abubakar yabo, Abdulrahman Ibrahim (PROFSTA). Abubakar Abdullahi (GWANKS), Aisha Abdulmalik who guide me with good advice and helps in so many things during this wonderful program. And my deepest gratitude to whom their names are so many to be mentioned thank you all and love you all.
TABLE OF CONTENT
Title page……… ……. ……. …….. ……. ……….. ……… ……. …… ……. ……….. ………i
Certification……… ………. ……… ……….. ……. …… …… ….. ……… ……. ……….…...ii
Dedication………. ……… ……. …….. ….. ……… ……….. ……… …… ….. ……………...iii
Acknowledgement………… …………. ……….. …….. ………. ………. …….. ……………..iv
Table of content……… ………… …………… ……….. ………….. ……….. ………………..v
List of table of content……… ……… …….. ……… ……….. ……….. ………… …………...vi
List of table of content……….. ……….. ……….. ……….. …………. …………. …………..vii
List of table ……… ………. ………. ……….. ………. ……….. …………………………….viii
Abstract …… ……… …….. ……… …… …….. …… ……. …… …… ….. …. ….. ……….. ix
Chapter one
Introduction……….. ………. ………. ……….. ……….. ………. ……… …………..1-4
1.1 Statement of the problem. ………. …….. ………… ………. ……….. ………. …….4-5
1.2 Aim and objectives of the study…. ………. …….. ………… ………. ……….. ………5
1.3 Significance of the study…. ………. …….. ………… ………. ……….. ………. ……..5
1.4 Scope and limitation of the study…. ………. …….. ………… ………. ……….. ……..5
1.5 Definition of some basic terms …. …………… ………. ………. …….. …….. ……...5-6
Chapter two
2.0 Literature review …….. ……… …….. ………… ……… ……….. …..... ……………..7
2.1 Introduction… ……..…….. ……… …….. ………… ……… ……….. ……...... ………7
2.2 Review of some related literature ………………. …….. ……… …….. ………… …17-13
Chapter three
3.0 Methodology…….. ……… …….. ………… ……… …………………… ……………14
3.1 Introduction…….. ……… …….. ….......... …………………………………………….14
3.2 Research Design……… …….. ………… ……… ……….. ……............... ……………14
3.3 Data Description …….. ……… …….. ………… ……… ……….. ………… .......14
3.4 Multivariate Variables Specification…….. ……… …….. ………… ……… ……14-16
3.5 Statistical Methodology…….. ……… …….. ………… ……… ……….. ……… ...16-18
3.6 Hypothesis Testing In MANOVA…….. ……… …….. ………… ……… ………..18-19
3.6.1 Pillai’s Trace…….. ……… …….. ………… ……… ……….. ……. .............. ……….19
3.6.2 Hotelling- Lawley’s Trace…….. ……… …….. ………… ……… ……….. ……........19
Wilk’s Lamda (… ……..…….. ……… …….. ………… ……… ……….. ……20-21
3.6.4 Roy’s Largest Root… … ……… ………. …….……………………………………….21
Statistical package to be used for the study….. ……… ……… ……… ………………22
3.8 Data description…….. ………. ………. ……… ……… …….. ……… ……. …… ….23
3.9 Data analysis…….. ………. ……… ……… ……… ……… …….. …….. …….. ...23-30
Chapter four
4.0 Summary conclusion and recommendation. …….. ……… …….. ……………………..31
4.1 Summary……………………….. ………………… ……………………………………31
4.2 conclusions…….. ……… …….. ………… ……… ……….. …….................................31
4.3 Recommendation. …….. ……… …….. ………… ……… ……….. ……................31-32
4.4 Reference…….. ……… …….. ………… ……… ……….. ……..............................33-39
List of table
Table 3.9.1: Bartlett Test of Homogeneity of Variance…….. ……. ……… ……… …………..23
Table 3.9.2: MANOVA for plots……… ……… ………. ………. ………. ……… …….. 23-24
Table 3.9.3: MANOVA for soil types….. ……… …….. ……… ……… …….. ……. ……24-25
Table 3.9.4: MANOVA for fertilizer types……. ………… ………… ………….. ………...25-26
Table 3.9.5: MANOVA for interaction between plots and soil types…………… …………….26
Table 3.9.6: MANOVA for interaction between plots and fertilizer types…………………..26-27
Table 3.9.7: MANOVA for interaction between soil types and fertilizer types….. ………...27-28
Table 3.9.8: MANOVA for interaction between plots, soil types, and fertilizer types………28-29
Table 3.9.9: General ANOVA for crop performance versus plots, soil, and fertilizer………29-30
ABSTRACT
This study carried out to determine the response of fertilizer types, plots and soil types on crop performance by using multivariate analysis of variance (MANOVA). The data regarding the field courtesy of Najanu memorial farms was collected and analyze based on multivariate statistics such as wilk`s lambda. Pillai`s trace. Hotelling lawley`s and Roy`s criteria Hence the result confirmed that fertilizer types and any interaction with fertilizer types has significant effect on the crop performance, there by rejecting the null hypothesis at specified level of significance , whereas the remaining factors and the interaction has no significance effect on the crop performance. Thus, this signifies that fertilizer types have strong impact on the performance of crops. Therefore, future researchers should carry out a similar research work with more than three interaction effects to compare the results and also find out among the fertilizer types specifically which one has more effect.
CHAPTER ONE
INTRODUCTION
This research work is based on the multivariate analysis of variance (MANOVA) which is an extension of univariate analysis of variance (ANOVA). In ANOVA, differences among various group means on a single response variable are studied. In MANOVA, the number of response variables is increased to two or more. The hypothesis concerns a comparison of vectors of group means. Using univariate analysis, multiple ANOVA’s were conducted independently. The research works topic of study is response of crop performance to fertilizer types, plots and soil type which can be suitable to conduct this research using MANOVA since there are dependent and independent variables. When only two groups are being compared, the results are identical to Hotelling’s T2 procedure. The multivariate extension of F-test is not completely direct. Instead, several test statistics are available, such as Wilks’ Lambda and Lawley’s. Everitt et al.,(1991)
Draft animals are often in poor condition at the end of dry season, and many extension programmers have recommended that farmers grow and conserve fodder for their draft animals. On-station trials have demonstrated the potential for growing forage legumes and pasture grasses, but, to date, the production of single-purpose fodder crops at farm-level in West Africa has been minimal. In southern Mali, small quantities of forage cowpeas have been grown, because this was made a condition of credit allocation (Sangare, et al., 1988). Even in this case there has been a tendency to make this a dual-purpose crop, with a small harvest for human consumption (Starkey, 1988d). Crops cultivation is the most important section of agriculture, and the other section is husbandry or animal rearing. Agriculture in Nigeria has greatly improved in the past years because of the advent of technology and other necessary infrastructure.
Initially, most Nigeria farmers merely engage two types of farming, first one is subsistence agriculture that is to provide food for their family usage in the very little scale, and the second one is the commercial agriculture that is when the farmer has a very large scale farming made available in the market. Growth in agricultural output has no doubt been on the rise as farmer are stepping away from subsistence agriculture and embracing modern civilization-investing in large scale farming and ultimately increasing agricultural products. Soil fertility replenishment should be considered as an investment in the natural resource capital (Sanchez et al., (1997). reversing soil fertility depletion is one of the requirements for increasing per capital agricultural production in Africa (Sanchez and Leakey, 1997). Options aimed at improving soil fertility should rely on soil nutrient – supplying capacity, available soil amendments, and judicious use of mineral fertilizers to achieve balance nutrient management systems. Such an approach is usually referred to as integrated soil fertility management (ISFM) and should be embedded in a framework that includes aspects such as: weather, the presence of 7 weeds, pests and diseases, crop management, and socio-economic aspects such as input and output prices and labor availability(Bontkes and Wopereis, 2003 a).
The Nigeria soil and climatic condition is very suitable for the production of wide verities of crops, there are over a hundred different food crops produced by farmers in Nigeria on yearly basis which includes rice, yam, maize, okra, millet, sorghum, beans, potatoes, cassava, carrot, onion garbage, cocoa, cocoa yam, pears, onion, vegetables and very many others. Therefore, whatever type of cultivation a farmer wants to conduct, the knowledge of fertilizer and the type of soil is the most important in determining the farmer outputs. Soil and fertilizer type are very significant factor in crop production. According to food and agriculture organization of the united nation Nigeria is the world number 1 producer of cassava because of the topography of the soil structure and texture; cassava farming has taken the center stage in Nigeria and contributes over 45 percent of Nigeria agricultural Gross Domestic production (GDP). Agriculture in Nigeria contributes merely 24 percent of the Nigeria total GDP, trailing behind petroleum which is the major Nigeria domestic product. Although Nigeria depends heavily on the oil industry for its budgetary revenue it is believed that if the agriculture sector is properly managed and enhanced, it would greatly boost the country’s gross domestic product and even replace oil on the list, considering the vast area of land that is unused in Nigeria.
In many African countries including Nigeria, the main use of fertilizer is on maize, sorghum/millet and rice (Camara et al., 2006) with cowpea receiving little attention from farmers in terms of fertilizer application. As farmers lack adequate nutrient resources to fertilize all crops, they prefer to apply fertilizers to cereals and rarely target fertilizers directly to grain legumes which are mostly grown on residual fertility (Zingora et al., 2008). It is generally believed by most cowpea growers that the production of legumes do not require inorganic fertilizer application (Kan’’ankuk’’a, 1999). This is due to excessive vegetation at expense of grain production of this crop under fertilized fields.
In 1990, it was speculated that about 82 million hectares out of Nigeria’s total land area of 91 million hectares were farmed. Much of this land was formed under the bush fallow system, a process whereby land is left idle for a period of time to allow natural generation of soil fertility and replacement of soil nutrient. It is believed that the agricultural sector is one of Nigeria’s potential sources of revenue that is yet underdeveloped and unexplored.
Although mineral fertilizers can improve crop nutrition, they are sparingly used by farmers in Nigeria, as in many regions in sub-saharan Africa, partly due to the prohibitive cost as a result of removal of government subsidies (Gerner et al., 1995). Sanginga et al., (2009) stated that fertilizer consumption pattern within nations in Africa are often sketchy and inconsistent. Most smallholder farmers in Africa use fertilizers, but they are seldom able to apply them at the recommended rates and at the appropriate time because of high cost, lack of credit, delivery delays, and low variable returns (Heisey et al., 1996). Such constraints are largely due to the lack of enabling policy environment in rural areas caused by poor road and market infrastructure typical in most African countries. The price of fertilizers in rural areas of Africa is usually at least twice the international price (Bumb et al, 1996). African countries subsidized fertilizers; however, the removal of fertilizers subsidies by most African governments has increased fertilizer prices in relation to crop prices in many of these countries (Bumb et al., 1996). Fertilizer recommendations disregard variations in crop demand and soil properties and farmers” access to inputs and commodity markets with scales that is too large to capture soil heterogeneity (Smaling et al., 2002). Farmers on their part lack of information about the best fertilizers to use for their particular fields and cropping practices, making the crop response to fertilizers more erratic and less profitable. Even within more localized recommendation domains, households operate at different stages of economic development leading to misuse and associated economic (chase et al., 1991) and environmental risks (Bundy et al., 2001).
STATEMENT OF THE PROBLEM
In Nigeria today, crop production has been largely inconsistent resulting to the lack of knowledge of the combination of soil type and fertilizer. An adequate knowledge on the right combination would help to increase crop production and maintain that for a long time. There is equally a concern by farmers and researchers on the both organic and inorganic fertilizers to be used to increase crop yield. Another problem of farmers is to know the relationship between soil type and crop yield.
Above all, this research work is highlights the response or effect of soil types and plots on crop performance, and also to find out whether fertilizer types affect the crop performance.
AIM AND OBJECTIVES OF THE STUDY
The aim of this research work is to investigate the response of crop to fertilizer types, plots and soil types. The above aim is achieved through the following objectives:
To test the homogeneity of variance among the variables (fertilizer types, plots and soil types) on crop performance.
To test for the response of fertilizer types, plots, and soil types on crop performance using multivariate tests.
To verify the variables that contribute more between soil types, plots, fertilizer type on crop performance using multivariate test.
SIGNIFICANCE OF THE STUDY
the importance of this research work is to enable both the farmers and the government to have clear view on whether effects of fertilizers and soil types really contribute to the performance of crop or not. The most importance of this study is mainly to find out if there is any relationship between fertilizer types and soil types on crop yield.
SCOPE AND LIMITATION OF THE STUDY
The scope of this study focus on crops performance to the types of soil and fertilizer used. The scope of this study is to find out whether fertilizer types, plots and soil types have impact in producing a best crop yield. There are many factors contributing the performance crops but due to the data collection, time and financial constraints, this study is limited to only knowing the response of fertilizer types, plots and soil types on the performance of crop.
DEFINITION OF SOME BASIC TERMS
Fertilizer: is the reason why plants grow. It also adds nutrients and texture to soil to provide nutrients some plants e.g. herbs, shrubs, flowers etc.
Organic fertilizer: is all natural and includes things such as bat, guano, compost, peat moss, wood ash and manure.
Inorganic fertilizer: this is primarily derived from chemical compound, either mineral or synthetic.
Crops: a plant that is grown in large quantities, especially as food.
Synthetic: this is a substances made artificial by combining chemical substances e.g. inorganic fertilizer rather than being produced by plants or animals which are the natural organisms e.g. organic fertilizer.
Soil: this is as a result of organic matter which coats soil particles, or can be define as the top layer of the earth surface, in which plants, trees etc.
CHAPTER TWO
2.0 Literature Review
2.1 Introduction
Multivariate analysis of variance (MANOVA) is simply an ANOVA with several dependent variables. This is to say, ANOVA test for the difference in means between two or more groups, while MANOVA test for the difference in two or more vectors of means. E.g. (Holloway et al.,1967).
For example, we may conduct a study where we try two different textbooks, and we are interested in the student’s improvements in statistics and physics. In that case, improvements in mathematics and physics are the two dependent variables, and our hypothesis is that both together are affected by the difference in textbooks. A multivariate analysis of variance (MANOVA) could be used to test this hypothesis. Instead of a univariate F-value, we would obtain a multivariate F-value (wilk’s ) based on a comparison of the error variance/covariance matrix and the effect variance/covariance matrix.
Although we only mention wilk’s here, there are other statistics that may be used, including hotelling’s trace and Pillai’s criterion. The “covariance” here is include because the two measures are probably correlated and we must take this correlation into account when performing the significance test.
Testing multiple dependent variables is accomplished by creating new dependent variables that maximize group differences. The artificial dependent variables are linear combinations of the measured dependent variables.
2.2 Review of Some Related Literature
In this chapter it emphases or highlight other related researches that have been made by other researchers using multivariate analysis of variance (MANOVA), to see how they come up with their ideas and conclusions, using method of multivariate.
Historically, the bulk of applications of multivariate techniques have been in the behavioral and biological science. However, interest in multivariate method has now spread to numerous other field of investigation. For example, I have collaborated on multivariate problems with researchers in education, chemistry, physics, geology, engineering, law, business, literature, religion, public broadcasting, nursing, mining, linguistics, biology, psychology, and many other fields.
Olusegun A. (2003) stated that 28% to 42% of nitrogen fertilizer applied to maize remained in the soil depending on nitrogen rate and tillage. Plassis L. (2003) found that 28% and 31% respectively, of urea and oxide nitrogen applied to sorghum were in the upper 25cm soil depth at the end of the cropping season.
Peters, et al., (1965) reported that soya beans yields increased from 1.3-1.9 t/ha when the nitrogen applications in the proceeding rice crop (residual effect) increased from 0 to 180kgN/ha. This means that the residual effect of nitrogen fertilization is affected by so many variables and is therefore site specific. The fertilizer rate applied, leaching, immobilization, DE nitrification and rainfall patterns are likely to affect the magnitude of the residual effect.
Ashalatha (1989) studies MANOVA for 17 genotypes with seven variables of Ragi at 15 locations. The results revealed that the genotype, location and GE-interactions were significant when tested against pooled error using wilk’s lambda criterion.
Ruìz-nogueira et al., (2001) field experiments were conducted on genotype x environment interaction and dry matter yield stability of white clover (Trifolium Opens l.) cultivars and breeding populations to study the dry matter yield peculiarities in 6 cultivars (Suduviai, Bitunai, Atoliai, Nemuniai, Milo and Gvendel) and 4 breeding populations (nos. 1123, 1124, 1421 and 1435) of white cover, ANOVA was employed to draw the consolations. Result of the ANOVA analysis showed that dry matter yield was essentially influenced by the cuts, year of trials, cultivars and their interactions. Promising breeding lines 1123 and 1124-combined high annual yield of dry matter with a low variance of stability (0.0593-0.0956).
Adebayo et al., (2014) field experiment was carried out at the teaching and research farm of Kwara state university, Malete, Ilorin, to evaluate the effect of compost, organ mineral, and inorganic fertilizers on the growth and yield of Amaranthus Caudatus as well as its residual effects. Amaranthus was growth with compost grade B (unlamented compost), organ mineral fertilizer Grade A (compost amended with mineral fertilizer). And NPK 15-15-15 and no fertilizer (control). All the treatments except control were applied at the rate of 100kgN/ha. The results indicated that Amaranthus yield of 18.9t/ha produced from grade A was significantly (P<0.05) higher than 17.6t/ha obtained from NPK fertilizer. Residual effect of Amaranthus growth parameters such as plant height , number of leaves, and yield values obtained from grade A was also significantly (P<0.05) higher than that of NPK, compost, and control values. Thus, organ mineral fertilizer could be used in cultivation of Amaranthus Caudatus in Ilorin and in similar type of soil in similar agro ecology.
Rachel et al., (2016) lonicera Maackii is an invasive Asian shrub naturalized in North America that has negative effects on forest understory vegetation. Aqueous extracts of leaves and roots of this plant have been shown to inhibit germination of several species. He examined the extent to which field-collected soils conditioned by L. Maackii growth, with and without additions of L. Maackii extracts, had effects on growth, morphology, and reproduction of Arabidopsis thaliana in a greenhouse setting. We also examine the effect of nutrient addition to the same soils on the performance of A. thaliana, whether plant responses to nutrients varied among L. Maackii- conditioned and unconditioned soils, and whether the effects of L. Maackii extracts varied in soils with different histories and fertility. Plant grown in forest soil collected from a site where L. Maackii was present grew slower and flowered later than plants grown in an unconditioned soil, but ended up being larger, with more leaves, branches and a higher total seed output. Nutrient addition had a positive effect on performance of A. thaliana, but when nutrients were added with extracts of L. Maackii roots and leaves, the positive effects of nutrient were greatly diminished. Inhibiting plant response to resource opportunities is a potentially important indirect effect of all alchemical. When direct effects of extracts on growth were seen, leaf extracts had a greater effect than root extracts. While the most important impacts of L.maackii in the field are undoubtedly due to competition for light and moisture, evidence for all allopathic effects of this plant is growing.
Zublena et al., (1990) earlier observed that poultry manure in crop husbandry must be done with sound soil fertility management practices to prevent soil nutrient imbalance and associated animal and human health risks, as well as surface-water and groundwater contamination. Therefore, addition of poultry manures soil for enhanced fertility must be done with caution.
Makind et al., (2010) earlier obtained similar increase in plant height of A. cruentus, though with increased organ mineral fertilizer applications in orthic luvisol and Dystric fluvisol soils gave similar results of increased leafy productivity of grain amaranth when the combination of poultry manure and urea-N fertilization was compared with conventional fertilizer application.
Alley and Vanlauwe (2009) defined soil fertility as the capacity of soil to retain, cycle and supply essential nutrients for plant growth over extended periods of time. It is important to crop production as the soil is the nutrient base for plants but low soil fertility, particularly N and P deficiencies, is one of the major biophysical constraints affecting African agriculture (Mokwunye et al., 1996). The import of these is that no matter how other factors of production are remedied, food production in Africa will continue to decrease unless there is conscious effort to address soil fertility depletion. Nutrient depletion rates are field specific, depending on the way each particular field has been managed over decades (Sanchez et al., 1997) hence the replenishment should also be field/site specific for effective results. Nutrient depletion rates vary with soil properties. The proportion of nutrient loss is normally greater in sandy soils because soil organic matter (SOM) particles are less protected from microbial decomposition in sandy soils than in loamy or clayey soils (swift et al., 1994).
Burow KR et al., (2003). In his study, he examined the influence of different nitrogen (N) application rates (0, 168, 240, 270 and 312 kg N ha-1) on soil properties, maize (Zea Mays L.) yield and microbial communities of three types of soils (clay, alluvial and sandy soils). Results indicated that N fertilization significantly decreased microbial biomass in both clay and sandy soils regardless of application rate. These decreased were more likely a result of soil pH decreases induced by N fertilization, especially in the sandy soils. This is supported by structural equation modeling and redundancy analysis results. Nitrogen fertilization also led to significant changes in soil microbial community composition. However, the change differences were gradually dismissed with increase in N application rate. We also observed that N fertilization increased maize yields to the same level regardless of application rate. This suggests that farmers could apply N fertilizers at a low rate (i.e. 168 kg N ha-1), which could achieve high maize yield on one hand while maintain soil microbial functions on the other hand.
Emmanuel (2014). The attendant low yield of cowpea among smallholder farmers has increased the need for site specific fertilizer recommendation and integration of biological materials to increase the yield of crop. The effectiveness of applied fertilizer is constrained by the use of the inappropriate rate and improper timing of sowing. Rhizobia Inoculant, on the other hand, needs a balanced nutrient application to increase crop yield. The study was designed to: i) determine the effect of inoculants, P and K mineral fertilizers on N, P, K uptake, growth and grain yield of cowpea, ii) evaluate the effect of NPK fertilizer application on the growth and yield of cowpea in the Guinea and Sudan savanna zones of Ghana, and iii) simulate the potential yield, yield gap, best sowing date, growth and yield of cowpea using CROPGRO cowpea DSSAT model. The response of cowpea to Rhizobia inoculation and fertilizer application revealed that sole application of inoculants was not sufficient to raise cowpea yield except when combined with 30 kg P205 ha-1 and 20 kg K20 ha-1. The yield response to mineral fertilizer also showed that K is very essential in determining cowpea yield and should therefore, not be omitted in any fertilizer recommendation rate. CROPGRO – cowpea model was used to simulate the response of cowpea to different N rates, potential yield and the best sowing dates for cowpea. The model was calibrated for Omondaw cowpea cultivar using data from the experiment carried out at Lawra (ferric Lixisol) and Nyoli (ferric Luvisol) during the 2012 and 2013 cropping seasons. The model performance was evaluated statistically using RMSE (0.13 tons ha-1), CV (RMSE) (9.9%) and Wilmott index of agreement d (0.97). A long term seasonal analysis using the model was able to detect that 15kg N ha-1 is optimal for ferric Lixisol while 20kg N ha-1 was optimal for ferric Luvisol.to avoid crop failure, sowing window for Lawra range from july 11 xvii to july 21st while that of Nyoli was extended to 26th July with consideration for august 5th. The yield gap analysis revealed that a wide gap exists between climatic potential yields and yields obtained from farmers’ fields. The gaps between potential and research station yields (yield gap 1) range from 1.05 to 18% of potential yield, while that of the farmer’s field was 84.21% for Lawra soil and 79.55% for Nyoli soil. It is necessary to reduce the yield gap by using site specific fertilizer recommendation and appropriate timing of sowing dates.
CHAPTER THREE
3.0 Methodology
3.1 Introduction
This chapter consists of method of data collection used for this study and method of data analysis. Method of data collection section gives details on how the data were collected and method of data analysis, and also in this section the explanation of the statistical tools and statistical software used for the analysis.
3.2 Research Design
This study focused on an investigation of what cause the other among different variables. This study is most preferred because the study will be investigation the response of crop to fertilizers and soil types. This study adopted both descriptive and explanatory research design. First, the study described the crop performance on soil and fertilizer types.
3.3 Data Description
In the course of this study, the data used for the analysis was strictly the secondary data. It was extracted from the research and record book of Najanu Memorial Farms Kaura Namoda Zamfara state.
3.4 Multivariate Variables Specification
The multivariate variables model for the study consists of three constructs as described below:
soil type
fertilizer type used
crop performance
Soil Type
Firstly, soil is as a result of organic matter which coats soil particles, Brady, N.C. (2000). The soil type used for this study consists of the following three types of soil:
loamy soil type
sandy soil type
clay soil type
Loamy Soil Type
Loamy soil is the most desirable soil texture for most plant cultivation. The loam class of soil presents the optimum habitat for plant health. It retains moisture without impeding drainage, captures nutrients and allows oxygen and microorganisms to circulate. There are different of loam soil, classified according to the composition of the soil particles contained in a sample. Jenny, Hans. (1999).
Sandy Soil
This is granular soil that contains small rock and mineral particles. The texture of sandy soil is usually gritty. This soil feel course whether it is dry or wet. Sandy soils are the result of the weathering and disintegration of a variety of rocks such as granite, limestone and quartz. This type of soil is easy to cultivate but, since it allows for more drainage than needed, it is important to water it regularly, especially during summer days. As sandy soil doesn’t allow the water to pool around the roots, they are a good choice for plants that have a tendency to suffer from root decay. Millar et al., (2002).
Clay Soil
This is a fine-grained natural rock or soil materials that combine one or more clay minerals with traces of metal oxides and organic matter. Clays are plastic due to their water content and become hard, brittle and non-plastic upon drying or firing. (Wikipedia the free encyclopedia, 2015)
Fertilizer Types
Fertilizer is an essential part of growing plants. It adds nutrients and texture to soil to provide nutrient to trees, vegetables, herbs, shrubs and flowers. There are several ways to categorize fertilizer, and the most basic is whether it is organic or inorganic.
Organic fertilizer
Inorganic fertilizer
Organic Fertilizer
Is all natural and includes things such as bat guano, compost, peat moss, wood ash and manure. Singer, et al.,(2000)
Inorganic Fertilizer
This is primarily derived from chemical compounds, either mineral or synthetic. They typically come as a powder, pellets, granules or a liquid. Most inorganic, concentrated fertilizer is rated based on the percentage of nitrogen, phosphorous and potassium. Other chemicals that might be included in inorganic fertilizers include calcium, sulfur, iron, zinc magnesium.
Crop Performance
The performance of crops was rated on a scale of 1 to 5. With 5 signifying crops that performed very well and 1 signifying poor performance of crops.
3.5 Statistical Methodology
The data to be analyzed consist of independent variables represented by the treatments accordingly: i.e. plots, soil types and fertilizers used (inorganic and organic) and the dependent variable i.e. the crop performance. We wish to observe the effects of treatment on the independent variables simultaneously.
MANOVA Advantages over ANOVA
It tests the effects of several independent variables and outcome (dependent) variables within a single analysis
Independent variables of interest are likely to affect a number of different conceptual variables - for example: in the case of this research topic.
Since only ‘one’ dependent variables is tested, the researcher is protected against inflating the type 1 error due to multiple comparisons.
Assumption Of Multivariate Analysis Of Variance (MANOVA)
In multivariate analysis of variance (MANOVA) the following assumptions are made when using a MANOVA. Wilks, S.(1946)
Normal Distribution
The dependent variable should be normally distributed within groups. Overall, the F test is robust to non-normality, if the non-normality is caused by skewness rather than by outliers.
Linearity
MANOVA assumes that there are linear relationships among all pairs of dependent variables in each cell. Therefore, when the relationship deviates from linearity, the power of the analysis will be compromised.
Homogeneity of Variances
Homogeneity of variances assumes that the dependent variables exhibit equal levels of variance across the range of predictor variables. Remember that the error variance is compute (Ss error) by adding up the sum of squares within each group. If the variances in the two groups are different from each other, then adding the two together is not appropriate, and will not yield an estimate of the common within-group variance.
Homogeneity of Variances and Covariances
In multivariate designs, with multiple dependent measures, the homogeneity of variance assumption described earlier also applies. There are various specific tests for this assumption has minimal impact if the groups are of approximately equal size. Therefore, every effort should be made to have equal cell sizes. (Alin and Kurt, 2006),
Significance Test of Multivariate Analysis of Variance (MANOVA)
In statistics, multivariate analysis of variance (MANOVA) is a procedure for comparing multivariate sample means. As a multivariate procedure, it is used when there are two or more dependent variables, and is typically followed by significance test involving individual dependent variables separately. It helps to answer so many questions one of which is:-
Do changes in the independent variable(s) have significant effects on the dependent variables?
3.6 Hypothesis Testing In MANOVA
The reason for four different statistics and for approximations is that the mathematics of MANOVA get so complicated in some cases that no one has ever been able to solve them. To understand MANOVA, it is not necessary to understand the derivation of the statistics. Here, all that is mentioned is their names and some properties.
The four most widely used measures for assessing significance between groups on the independent variables are:
1. Pillai’s Trace
Hotelling- lawley Trace
Wilk’s Lambda (
Roy’s Largest Root
3.6.1Pillai’s Trace
The Pillai’s-Bartlett trace is the sum of explained variances on the discriminant variates , which are the variables which are computed based on the canonical coefficients for a given root. The larger the Pillai’s trace, the more the given effect contributes to the model. Pillai’s trace is always smaller than Hotelling’s trace. This is the most robust test of all each measure/test is preferred in differing situations. Pillai, K.C.S. (1964)
Pillai’s criterion is considered more robust and should be used if sample size decreases, unequal cell sizes appear or homogeneity of covariance is violated. Some statisticians consider it to be the most powerful and most robust (type I sense) of the four statistics. An alternative criterion for testing the null hypothesis H0: = … , was proposed by Pillai’ K.C.S et al., (1965). The formula for the Pillai’s trace is given as:-
Pillai’s trace: V2 = (3.1)
Hotelling- Lawley’s Trace
It is a test to assess the statistical significance on the difference of the means of two or more variables between the groups. It is special case of MANOVA used with two groups of a treatment variable. The larger the Hotelling’s trace, the more the given effect contributes to the mode. Hotelling, H. (1951) is defined as
Hotelling’s trace: T = (3.2)
Wilk’s Lamda (
Wilk’s lambda is a test statistics used in multivariate analysis of variance (MANOVA) to test whether there are differences between the means of identified groups of subjects on a combination of dependent variables. For example, we have to test whether the mean score of two groups, graduates and diplomats, is the same across eight constructs simultaneously. Thus, there are eight dependent variables and comparing the mean of this combination for two groups. Wilk’s lambda performs, in the multivariate setting with a combination of dependent variables, the same role as f-test performs, in one-way analysis of variance. Wilk’s lambda is a direct measure of the proportion of variance in the combination of dependent variables that is unaccounted for by the independent variable (the grouping variable or factor). If a large proportion of the variance accounted for by the independent variable then it suggests that there is an effect from the grouping variable and that the groups (in this case the graduates and diplomats) have different mean values. The t-test, Hotelling’s T, and F-test are special cases of wilk’s lamda. Wilk’s lamda ranges from 0 to 1 and the lower the wilk’s lamda, the more the given effect contributes to the model.
Wilk’s was the first manova test statistic developed and is very important for several multivariate procedures in addition to manova. It was proposed by s.s wilk’s (1906-1964). This statisticwilk’s (1932), which is often called the wilk’s which may be defined as the ratios of the two determinants (variance) of S matrices are found. Wilk’s the test statistic preferred for MANOVA, and is found through a ratio of the determinants
Mathematically as:
(3.3)
With e
The following approximation based on the f-distribution is used to determine significance levels.
We reject H if Note that the rejection is for small values of Wilk’s. The parameters in wilk’s
p= number of variables (dimension)
h= degree of freedom for hypothesis
e= degree of freedom for error.
The quantity (1- is often interpreted as the proportion of variance in the dependent variables explained the model effect. However, this quantity is not unbiased and can be quite misleading in small samples.
Determination involves finding functions of matrix called eigenvalues. The matrix of interest for the Wilk’s is actually product of two matrices E-1H, where the matrices, E and H, are as described earlier. The eigenvalues are obtained by solving the determinant equation -1H – Thus, wilk’s
Wilk’s lambda: ) (3.4)
The number of nonzero eigenvalues of E-1H is q = min (p,h) which is the rank of the H. The matrix HE-1 has the same eigenvalues as HE-1 and could be used in its place to obtain however we prefer HE-1 because we use it in eigenvectors.
Roy’s Largest Root
This test measures the differences on only the first discriminant function among the dependent variables. This criterion provides advantages in power and specificity of the test but makes it less useful in certain situations where all dimensions should be considered. This test is more appropriate when dependent variables are strongly interrelated on a single dimension, but it is also the measure most be severely affected by violations of the assumptions. This is often test for H0: = = …. = based on we use Roy’s union-intersection the test statistic is computed as:
Roy’s largest root: (3.5)
The maximum eigenvalue of A-1 (Recall that a “root” is another name for an eigenvalues) Hence, this statistic could also be called Roy’s largest eigenvalue. And eigenvectors of -1
In some cases, the four will generate identical F statistics and identical probabilities. In other’s they will differ. When they differ, Pillai’s trace is often used because it is the most powerful and robust (type I sense). Because Roy’s largest root is an upper bound on f, it will gives lower bound estimate of the others are not significant.
Generally, if group sizes are equal, the tests are sufficiently robust with respect to heterogeneity of covariance matrices so that we need not worry. If the ni’s are unequal and we have heterogeneity, then the -level of the manova test may be affected as follows. If the larger variance and covariance are associated with the larger samples, the true -level is reduced and the result becomes conservative. On the other hand, if the larger variance and covariance come from smaller samples, is inflated, and the tests become liberal.
Statistical Package To Be Used For The Study
MANOVA analysis can be conducted by using several statistical package or soft wares but in the case of this research work or project MINITAB is the statistical software to be used for analysis of this research work. And also for homogeneity of variance R-package was used to see if the homogeneity among the variance exists.
Data Description
The data collected on the crop performance base on the soil types and different types of fertilizer being into two different types of plots, in which organic fertilizer is applied to plot one and inorganic fertilizer is applied to plot two
Plots
Soil types
Fertilizer types
Crop performance
Plot1
Plot2
Loamy
Sandy
Clay
Organic
Inorganic
Very Poor
Poor
Fair
Well
Very Well
1
2
1
2
3
1
2
1
2
3
4
5
Data Analysis
Table 3.9.1: Bartlett Test of Homogeneity of Variance
Test
DF
p-value
Bartlett’s
33.055
3
3.135e-07
From the above table the p-value (3.135e-07) is less than the value of alpha (0.05), we therefore reject the null hypothesis and conclude that the homogeneity among the variance exist.
Table 3.9.2: MANOVA for plots
Criterion
Test Statistic
F
DF
P
Wilk’s
0.89888
0.900
(1, 8)
0.371
Lawley-Hotelling
0.11250
0.900
(1, 8)
0.371
Pillai’s
0.10112
0.900
(1, 8)
0.371
Roy’s
0.11250
From the table 3.9.2.Above, we have the following conclusions;
Decision: the p-value (0.371) corresponding to the plots is greater than the value of alpha (0.05). Therefore, we accept the null hypothesis
Wilk’s lambda ( test: from this test we concluded that plots factor has significant effect on crop performance at 5% level of significance.
Lawley-Hotelling test: this test testified us that the means between the crop performance and plots does not differ significantly i.e. is equal at 5% level of significance.
Pillai’s test: this test shows us that the relationship that exists between the crop performance and plots is significantly least at 5% level of significance.
Roy’s test: it testified that plots factor has no significant effect on crop performance when the remaining independent variables (fertilizer and soil types) were not considered at 5% level of significance.
Table 3.9.3: MANOVA for soil types
Criterion
Test Statistic
F
DF
P
Wilk’s
0.84325
0.744
(2, 8)
0.506
Lawley-Hotelling
0.18588
0.744
(2, 8)
0.506
Pillai’s
0.15675
0.744
(2, 8)
0.506
Roy’s
0.18588
From the table 3.9.3 above, we have the following conclusions;
Decision: the p-value (0.506) corresponding to the soil types is greater than the value of alpha (0.05). Therefore, we accept the null hypothesis
Wilk’s lambda ( test: from this test we deduced that the soil types have no significant effect on crop performance at 5% of significance.
Lawley-Hotelling test: this test testified us that the means between the crop performance and soil types does not differ significantly i.e. is equal at 5% level of significance.
Pillai’s test: this test shows us that the relationship that exists between the crop performance and soil types is significantly least at 5% level of significance.
Roy’s test: it testified that soil types have no significant effect on crop performance when the remaining independents variables (fertilizer and plots) were not considered at 5% level of significance.
Table 3.9.4: MANOVA for fertilizer types
Criterion
Test Statistic
F
DF
P
Wilk’s
0.11348
62.500
(1, 8)
0.000
Lawley-Hotelling
7.81250
62.500
(1, 8)
0.000
Pillai’s
0.88652
62.500
(1, 8)
0.000
Roy’s
7.81250
From the table 3.9.4 above, we have the following conclusions;
Decision: the p-value (0.000) corresponding to the fertilizer types is less than the value of alpha (0.05). Therefore, we reject the null hypothesis
Wilk’s lambda ( test: from this test we observed that the fertilizer types have significant effect on crop performance at 5% level of significance.
Lawley-Hotelling test: this test testified us that means between the crop performance and fertilizer types does differ significantly i.e. is not equal at 5% level of significance.
Roy’s test: it testified that fertilizer types have significant effect on crop performance when the remaining independents variables (soil types and plots) were not considered at 5% level of significance.
Table 3.9.5: MANOVA for interaction between plots and soil types
Criterion
Test Statistic
F
DF
P
Wilk’s
0.66824
1.986
(2, 8)
0.199
Lawley-Hotelling
0.49647
1.986
(2, 8)
0.199
Pillai’s
0.33176
1.986
(2, 8)
0.199
Roy’s
0.49647
From the table 3.9.5 above, we have the following conclusions;
Decision: the p-value (0.199) corresponding to the interaction of plots and soil types is greater than the value of alpha (0.05). Therefore, we accept the null hypothesis
Wlik’s lambda test: from this test we concluded that the interaction of plots and soil types has no significant effect on crop performance at 5% level of significance.
Lawley-hotelling test: this test testified us that the means between the crop performance and the interaction of plots and soil types does not differ significantly i.e. is equal at 5% level of significance.
Pillai’s test: this test shows us that the relationship that exists between the crop performance and the interaction of plots and soil types is significantly least at 5% level of significance.
Roy’s test: it testified that the interaction of plots and soil types have no significant effect on crop performance when the remaining independent variable (fertilizer) was not considered at 5% level of significance.
Table 3.9.6: MANOVA for interaction between plots and fertilizer types
Criterion
Test Statistic
F
DF
P
Wilk’s
0.62016
4.900
(1, 8)
0.058
Lawley-Hotelling
0.61250
4.900
(1, 8)
0.058
Pillai’s
0.37984
4.900
(1, 8)
0.058
Roy’s
0.61250
From the table 3.9.6 above, we have the following conclusions;
Decision: the p-value (0.058) corresponding to the interaction of fertilizer types and plots is less than the value of alpha (0.1). Therefore, we reject the null hypothesis
Wlik’s lambda ( test: from this test we observed that the interaction of fertilizer types and plots has significant effect on crop performance at 10% level of significance.
Lawley-Hotelling test: this test testified us that the means between the crop performance and that of interaction between fertilizer types and plots does differ significantly i.e. is not equal at 10% level of significance.
Pillai’s test: this test shows us that the relationship that exists between the crop performance and the interaction of plots and fertilizer types is significantly high at 10% level of significance.
Roy’s test: it testified that the interaction of plots and fertilizer types has significant effect on crop performance when the remaining independent variable (soil types) was not considered at 10% level of significance.
Table 3.9.7: MANOVA for interaction between soil types and fertilizer types
Criterion
Test Statistic
F
DF
P
Wilk’s
0.52861
3.567
(2, 8)
0.078
Lawley-Hotelling
0.89176
3.567
(2, 8)
0.078
Pillai’s
0.47139
3.567
(2, 8)
0.078
Roy’s
0.89176
From the table 3.9.7 above, we have the following conclusions;
Decision: the p-value (0.078) corresponding to the interaction of fertilizer types and soil types is less than the value of alpha (0.1). Therefore, we reject the null hypothesis
Wlik’s lambda ( test: from this test we observe that the interaction of fertilizer types and soil types has significant effect on crop performance at 10% level of significance.
Lawley-Hotelling test: this test testified us that the means between the crop performance and that of interaction between fertilizer types and soil types does differ significantly i.e. is not equal at 10% level of significance.
Pillai’s test: this test shows us that the relationship that exists between the crop performance and the interaction of fertilizer types and soil types is significantly high at 10% level of significance.
Roy’s test: it testified that interaction of soil types and fertilizer types has significant effect on crop performance when the remaining independent variable (plots) was not considered at 10% level of significance.
Table 3.9.8: MANOVA for interaction between plots, soil types, and fertilizer types
Criterion
Test Statistic
F
DF
P
Wilk’s
0.55921
3.153
(2, 8)
0.098
Lawley-Hotelling
0.78824
3.153
(2, 8)
0.098
Pillai’s
0.44079
3.153
(2, 8)
0.098
Roy’s
0.78824
From the table 3.9.8 above, we have the following conclusions;
Decision: the p-value (0.098) corresponding to the interaction of three independent factors is less than the value of alpha (0.1). Therefore, we reject the null hypothesis
Wlik’s lambda ( test: from this test we observed that the interaction of three independent factors has significant effect on crop performance at 10% level of significance.
Lawley-Hotelling test: this test testified us that the means between the crop performance and that of interaction between the three independent factors does differ significantly i.e. is not equal at 10% level of significance.
Pillai’s test: this test shows us the relationship that exists between the crop performance and the interaction of three independent factors is significantly high at 10% level of significance.
Roy’s test: it testified that the interaction of soil types, plots and fertilizer types have significant effect on crop performance at 10% level of significance.
Table 3.9.9: General ANOVA for crop performance versus plots, soil, and fertilizer
Source
DF
S.S
MS
F
P
Plots
1
5.8814
5.8814
4.0044
0.371
Soil types
2
4.0512
2.0256
1.37913
0.506
Fertilizer types
1
38.8687
38.8687
26.4638
0.000
Plots and soil types
2
0.2325
0.11625
0.07914
0.199
Plots and fertilizer types
1
0.9259
0.9259
0.6304
0.058
Soil types and fertilizer types
2
5.0000
2.5000
1.70213
0.078
Plots, soil types, and fertilizer types
2
0.0000
0.0000
0.0000
0.098
Error
8
11.7500
1.46875
Total
19
66.7097
The table 3.9.9 above shows the analysis of variance for the crop performance versus plots, soil types and fertilizer types. Hence the result confirmed the earlier discussion above that fertilizer types and any interaction with fertilizer types has significant effect on the crop performance, there by rejecting the null hypothesis at specified level of significance, whereas the remaining factors and interaction has no significant effect on the crop performance. Thus, this signifies that fertilizer types have a strong impact on the performance of crops.
CHAPTER FOUR
4.0 SUMMARY, CONCLUSION AND RECOMMENDATION
4.1 Summary
The project work titled multivariate analysis of variance (MANOVA) on the response of crop performance to fertilizer types, plots and soil types. This project reflects on how it has met its target aims and objectives, stated its statement of the research problem, significance of the study, scope and limitation.
Related literature were reviewed, source of case study, method of data collection, method of data analysis which use the MINITAB and R-package for the analysis. The analysis which included test of homogeneity of variance using Bartlett’s test, conclusion and recommendation were made
4.2 Conclusions
From the view of the above chapter three interpretations of results the following conclusions can be observed:
The result obtained from the Bartlett’s test of homogeneity or equality of variance shows that there is equality of dependent variable across the group at 5% level of significance. And from the multivariate analysis result we observed that the fertilizer types and any interaction with fertilizer types has significant effect on the crop performance, there by rejecting the null hypothesis at specified level of significance, whereas the remaining factors and interaction has no significant effect on the crop performance. Thus, this signifies that fertilizer types have a strong impact on the performance of crop; this was even justified from the ANOVA result.
4.3 Recommendations
1. Future researchers should carry out similar research work with more than three interaction effect to compare the results.
2. It is recommended if the future research focused on finding out what fertilizer type is suit soil best.
3. Moreover, the future research can also find out the best combination between soil types and specific crop.
4. It is advisably to farmer to adopt using both fertilizer types more especially applying organic first before inorganic fertilizer because organic helps inorganic in given a better yield.
REFERENCES
Alin and Kurt S. (2006). Statistical methods in medical research”.
Alley, M.M. and Vanlauwe, B. (2009). “The role of fertilizers in integrated plant nutrient
management First edition”, IFA, Paris, France, TSBF-CIAT, Nairobi Kenya.
Anaike, O.E. (2008). A project work on two-way MANOVA with interaction in physical
science of imo state university, Owerri, Department of sciences. Unpublished B.Sc project.
Ashalatha KV., (1989). A statistical Appraisal of Genotype-Environment interactions in Ragi
(Eleusine Coracana, G) under multicocation trails. M.Sc (agric), thesis university of Agricultural Sciences, Bangalore (India).
Bontkes, T.E.S and Wopereis, M.C.S (2003). Perspectives for the use of decision support tools
in agricultural research and development in Sub-Sahara Africa, pp. 150-158. in T.E Struif Bontkes and Wopereis, M.Sc (eds.) decision support tools for smallholder agriculture in sub-Sahara Africa a practical guide. IFDC and CTA.
Brady, N.C (2000). The nature and properties of soil 10th Ed. New York: Mcmillan.
Bumb, B.l.and Baanante, C.A. (1996). The role of fertilizers in sustaining food security and
protecting the environment to 2020. Food, Agric. And the Environ. Dis. Pap. 17. Int. food policy res. Inst., Washinton, DC.
Bundy, l.G., Andraski, T.W. and Powell, J.M. (2001). Management effects on phosphorus
losses in runoff in corn production systems. Journal of environmental quality, 30: 1822-1828. 131.
Burow KR, Nolan BT, Rupert MG, Dubrovsky NM. (1991-2003) nitrate in groundwater of
the united states,. Environ sci technol. 2010; 44: 4988-4997. DOI: 10.1021/es100546y [Pubmed].
Camara O, and Heinemann, E.D. (2006). overview of fertilizer situation in africa, 27 pp. in
proceedings of the African fertilizer summit. June 9-13, 2006, Abuja , Nigeria.
Chase, C., Duffy, M., Webb, J. and Vosss, R.(1991). An economic assessment of maintaining
High phosphorus and potassium soil test levels. American journal of alternative Agriculture 6: 83-86.
Emmanuel Obianuju Chiamaka. (2014) “a thesis presented to the department of crop and soil
sciences, faculty of agriculture, college of agriculture and natural resources, kwame Nkrumah university of science and technology, Kumasi Ghana, in partial fulfillment of the requirements for the award of degree of doctor of philosophy in soil sciences”.
Everitt B.S & Dunn G. (1991) applied multivariate data analysis. Edward Arnold. London.pp. 219-220.
Gerner, H., Asante, E.O., Owusu Bennoah, E. and Marfo, K.(1995). Ghana fertilizer
privatization scheme. Private sector role and public sector responsibilities in meeting needs of farmers. IFDC- Africa, Lome Togo.
Heisey, P.W. and Mwangi, W. (1996). Fertilizer use and maize production in sub-Sahara
Africa. Econ. Work. Pap. 96-01. CIMMYT, Mexico City.
Holloway, l.N. and Dunn, O.J (1967) the robustness of Hotelling T2. Journal of the America
statistical association, 62, 124-136.
Hotelling, H. (1951), “the generalization of student’s ratio,” annals of mathematical statistics, 2, 360-378
Jenny, Hans. (1999). Factors of soil formation; a system of quantitative pedology. (originally
published by mcgraw-hill; mineola, n.y.dover).
Kan’ankuk’a, C.N. (1999). Effect of lime, n and p on growth, yield and yield components of
cowpea (v.unguiculata [l] walp.). thesis submitted to the postgraduate school, Ahmadu Bello University, Zaria, 110 pp.
Makinde, E.A., l.S., Ojeniyi, S.O. 2010. Morphological characteristic of Amaranthus cruentus l. as influenced by kola pod husk, organomineral and NPK fertilizers in southwestern Nigeria. New York science journal 3(5): 130-134.
Millar, C.E., and Turk, l.M. (2002). Fundamentals of soil science. New Delhi: Biotech Books.
Mokwunye, A.U., de Jager, A. and Smaling, E.M.A. (1996). Restoring and maintaining the
productivity of West African soils: key to sustainable development. Misc fert. Stud. 14. International fertilizer development centre (IFDC)- Africa , Lome, Togo.
OLowoake Adebayo Abayomi and Ojo James Adebayo (2014) development of crop
production, Kwara State University, PMB 1530, Ilorin, Nigeria correspondence should be addressed to Olowoake Adebayo Abayomi; aolowoake@yahoo.com received 10 September 2014; revised 9 November 2014; accepted 10 November 2014; published 20 November 2014 academic editor: albino Maggio copyright © 2014 o. Adebayo Abayomi and O. James Adeb
Pillai, K.C.S. (1964), “on the distribution of the largest of seven roots of a matrix in multivariate
analysis,” biometrika, 51,270-275.
Pillai, K.C.S. (1965), “On the distribution of the largest characteristic root of a matrix in
multivariate analysis,” Biometrika, 52, 405-414.
Rachel E. Mcneishand Ryan W. Mcewan. (2016) a review on the invasion ecology of amur
honeysuckle (Loniceramaackii, Caprifoliaceae) a case study of ecology impacts at multiple scales. The journal of the Torrey botanical society 143:4, 367-385. Online publication date: 1-aug-2016.
Ruiz- Nogueira, B., Boote, K.J. and SAU, F (2001). Calibration and use of cropgro-soybean
model for improving soybean management under Rain fed conditions. Agricultural system 68(2): 151-173
Sanchez, P.A and Leakey, R.R.B., (1997). Land use transformation in Africa: three
determinants for balancing food security with natural resource utilization. European journal of agronomy 7:1-9.
Sanchez, P.A., Buresh, R.J and Leakey, R.R.B. (1997). Trees, soils, and food security
philosophical transactions of the royal society London, biological sciences 352 (1356): 949-961.
Sangaré M.I., Ladrette C.,Mungroop RR and Berthé A. 1988. Contraintes et ameliorations
de la traction animale en mali-sud:l’experience de la DRSPR pp. 191-211 in: P.H. Starkey and F. Ndiamé (eds), animal power in farming systems. Proceedings of network shop held 17-26 September 1986 in Freetown, Sierra Leone. Vieweg for German appropriate technology exchange, GTZ, Eschborn, Federal Republic of Germany. 363p. (e/f).
Sanginga, N. and Woomer, P.l. (2009). Integrated soil fertility management in Africa:
principles practices and development process. Tropical soil biology and fertility institute of the international centre for tropical agriculture. Nairobi. 263, pp.
Singer, M.J., and Munns, D.N. (2000). Soils: an introduction. USA: prentice-hall, Inc.
Smaling. E.M.A., stoorvogel, J.J., de Jager, A. (2002). Decision making on integrated nutrient
management through the eyes of scientist, the land user and the policy maker, pp. 265-284. In Vanlauwe, B., Diels, J., Sanginga, N. and Merckx, R. (Eds.), integrated plant nutrient management in sub-Sahara Africa. from concepts to practice. CAB international, Wallingford, Oxon, UK.
Starkey P.H 1988d.animal traction research in southern mali. Consultancy report for division de recherché Surles Systemses de Production Rurale (DRSPR), Sikasso, Mali. 30p. (e,f). (Unpublished).
Swift, M.J., Seward, P.D., Frost, P.G.H., Qureshi, J.N. and Muchena, F.N. (1994). Long-
term experiments in developing a database for sustainable land use under global change, pp. 229-251. In R.A. Leing and A.E. Johnson (eds.) long-term experiments in agricultural and agricultural and ecological sciences. Cab int., Wallingford, England.
Wall, F.J. (1967), “the generalize variance ratio or u-statistic”, Albuquerque, N.M.
White S., 1985. The Bauchi State Agricultural Development Project. Draught animal news
(centre of tropical veterinary medicine, Edinburgh) no. 4:17-20. (E).
Wilks, S.S. (1932), “certain generalizations in the analysis of variance”, Biometrika, 24, 471-
494.
Wilks, S.S (1946), “sample criteria for testing equality of means, equality of variances and
equality of covariances in a normal multivariate distribution”, annals of mathematical statistics, 17, 257-281.
Wilks, S.S. (1963), “multivariate statistical outliers”, Sankhya, series A, 25, 407-426.
Zingore, S., Murwira, H.K., Delve, R.J. and Giller, K.E. (2008). Variable grain legume
yields, responses to phosphorus and rotational effects on maize across soil fertility gradients on African smallholder farms. Nutr cycl agroecosyst 80: 1-18.
Zublena, J.P., Barker J.C., Carter T.A. 1990. Soil facts: poultry manure as a fertilizer source.
North Carolina extension services, publication A G-439-5.
0 Response to "A MULTIVARIATE ANALYSIS OF VARIANCE (MANOVA) ON THE RESPONSE OF CROP PERFORMANCE TO FERTILIZER TYPES, PLOTS AND SOIL TYPES (A CASE STUDY OF NAJANU MEMORIAL FARMS KAURA NAMODA ZAMFARA STATE) "
Post a Comment